The Human Layer of Laundering: Why Social Engineering Scams Are Escalating Across ASEAN
Social engineering scams are no longer fringe — they’ve become the engine of modern fraud and laundering across Southeast Asia.
From fake investment advisors to impersonated bank officials, these scams prey on human instincts — fear, trust, and urgency — to trick people into willingly moving illicit funds. And it’s working. In Malaysia alone, RM54 billion was reportedly lost to scams. Across the Philippines, call centres have been exposed for orchestrating high-volume fraud operations. Singapore faces rising impersonation cases, with victims transferring funds to seemingly legitimate local accounts.
Yet while the headlines focus on victims, AML teams face a different problem: these transactions often look clean on paper. The person transferring money isn’t a criminal — they’re a victim. The destination account may pass KYC, but it’s a mule. And the laundering happens not through loopholes in controls, but through the manipulation of people.
This blog explores why social engineering scams are a growing blind spot for AML professionals, how to detect the signs buried in behavioural and transactional data, and what institutions can do to adapt. Because when the crime starts with trust, detection must start with understanding intent.
{{cta-first}}
What Are Social Engineering Scams?
Social engineering scams involve psychological manipulation to deceive individuals into revealing confidential information or transferring money. These scams thrive not on system weaknesses, but on human vulnerabilities.
Common typologies include:
- Phishing: Fraudulent emails that mimic trusted entities
- Vishing: Voice-based scams using fake bank or authority impersonations
- Smishing: SMS-based fraud that incites urgency
- Pig-butchering scams: Long-term trust-building followed by a large financial con
- Deepfake impersonation: AI-generated audio or video to mimic real people
The sophistication of these scams has grown exponentially, especially with the rise of scam-as-a-service networks that rent out scripts, websites, and even call centre agents to fraudsters.

Why Social Engineering Is Booming in Southeast Asia
Southeast Asia is fertile ground for social engineering scams due to a perfect storm of technological access, financial inclusion, and regulatory gaps:
1. Digital Payments = Real-Time Risk
Instant payments and e-wallets have surged in adoption across ASEAN — with convenience comes irreversible transfers.
2. Trust in Authority Exploited
Scammers impersonate police, bank officials, or delivery agents. Cultural respect for authority makes victims more susceptible.
3. Social Platforms as Scam Channels
Telegram groups, Facebook job posts, and WhatsApp blasts are now tools for both recruitment and deception.
Recent Cases:
- Malaysia: 208,000+ mule accounts linked to students, job scams offering RM1,000 per account
- Philippines: “Love scam” call centres targeting foreigners, doubling as laundering hubs
- Singapore: Spike in fake bank alerts via SMS and WhatsApp
The Blind Spot in AML Programmes: Human Risk
Traditional AML systems are designed to detect anomalies in structured data — but social engineering operates in the shadows of human behaviour.
Key Gaps:
- ✅ Transactions appear “consented”: No flagged IPs, no breached credentials
- ✅ Victims appear compliant: They're coached on what to say if questioned
- ✅ Mule accounts pass KYC: Often opened legally by students or gig workers
- ✅ Siloed fraud and AML teams: Investigation teams miss behavioural patterns that started with fraud, not laundering
The result? Many scam-linked transfers never trigger alerts — or are dismissed due to a lack of clear suspicion.
How AML Teams Can Detect Social Engineering-Linked Transactions
It’s time for a layered approach — blending behavioural monitoring, linguistic cues, and typology intelligence.
Layer 1: Behavioural Red Flags
- First-time cross-border transfers
- Night-time or urgent fund transfers
- Drastic change in transaction volume
Layer 2: Transaction Narrative Clues
- Keywords like “emergency,” “loan,” “help,” “investment,” “crypto,” “family hospital”
- Inconsistent narrative vs. customer profile (e.g., student sending high-value remittance)
Layer 3: Pattern Matching Across Accounts
- Multiple unrelated users sending to the same beneficiary
- Similar message structures or amounts in scam campaigns
Layer 4: Shared Typologies and Intelligence
- Use shared scenario libraries (like the AFC Ecosystem) to detect evolving scams
- Community-shared red flags strengthen real-time detection coverage
What Institutions Can Do Now
1. Unify Fraud and AML Functions
Blur the lines between fraud and AML — scams often start as fraud and end as laundering.
2. Add Scam Typologies into Monitoring Scenarios
Include real-world scam narratives in detection logic: investment fraud, job scams, love scams, phishing-induced money mules.
3. Use AI to Read Between the Lines
Natural language models (LLMs) can analyse transaction narratives and customer communications for risk indicators.
4. Educate Customers & Staff
Proactive alerts, in-app scam warnings, and staff training on social engineering scenarios can intercept scams earlier.
5. Leverage Collective Intelligence
Join industry-wide ecosystems to stay updated on new scam formats, red flags, and fraud networks — no institution can fight this alone.
{{cta-whitepaper}}
Conclusion: When Scams Start with Trust, Detection Must Start with Intent
Social engineering scams have redefined the frontlines of financial crime. They target emotions, not systems, and as a result, bypass the traditional controls AML teams rely on.
For compliance professionals, this means a shift in mindset. It’s no longer just about tracing dirty money — it’s about recognising when legitimate money is moved under illegitimate influence.
AML frameworks must evolve to detect the unseen: the coercion, the manipulation, the trust betrayed. Because in a world where the victim is the money mule, and the scam is the method of laundering, the human layer is the new battleground.
Tookitaki’s FinCense platform is designed for this new reality — combining advanced AI, real-world scam typologies, and federated learning to surface hidden risks faster. By applying behavioural logic and collaborative intelligence, FinCense helps institutions detect scam-linked laundering in real time, without relying solely on static rules. It’s a smarter, more adaptive defence against the human side of financial crime.
Experience the most intelligent AML and fraud prevention platform
Experience the most intelligent AML and fraud prevention platform
Experience the most intelligent AML and fraud prevention platform
Top AML Scenarios in ASEAN

The Role of AML Software in Compliance

The Role of AML Software in Compliance

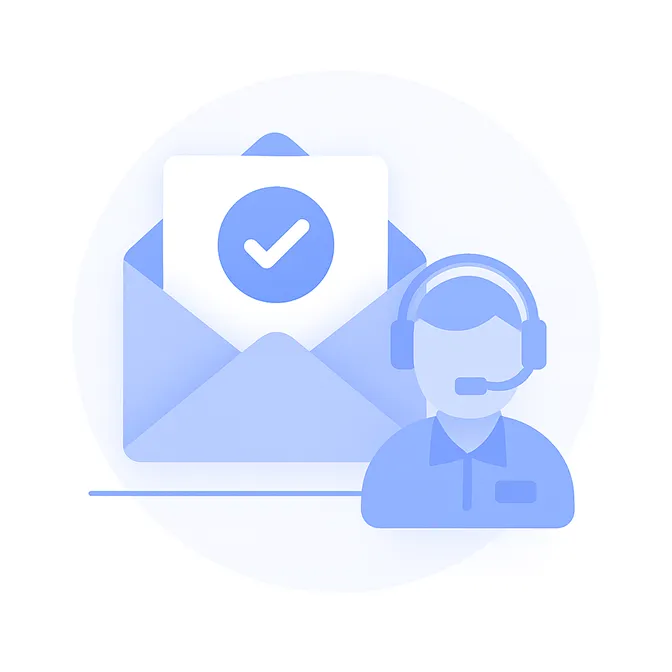
We’ve received your details and our team will be in touch shortly.
Ready to Streamline Your Anti-Financial Crime Compliance?
Our Thought Leadership Guides
Beyond Alerts: How AML Investigation Tools Are Transforming Compliance Operations
Financial crime is evolving—and your investigation tools should too.
As regulatory pressure intensifies and criminal tactics grow more sophisticated, the ability to investigate money laundering efficiently has become mission-critical for financial institutions. Traditional approaches often fall short—burdened by fragmented data, alert fatigue, and manual processes.
In this blog, we explore how modern AML investigation tools empower compliance teams with smarter workflows, real-time insights, and collaborative intelligence. From case management to behavioural analytics, we’ll unpack the features that matter most—and how to choose the right tools for your institution.
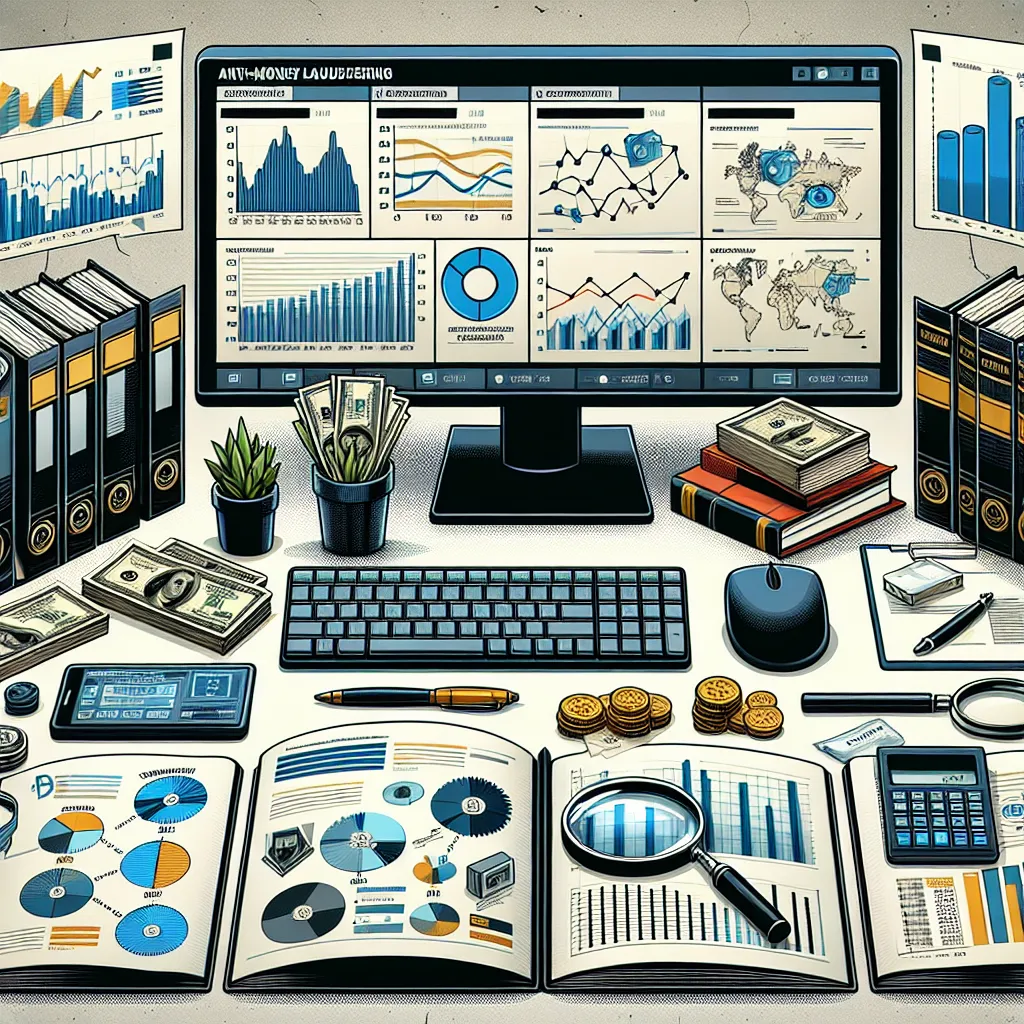
Understanding AML and Its Significance in Financial Crime Prevention
Anti-Money Laundering, commonly known as AML, refers to a set of laws and procedures. These are designed to prevent criminals from disguising illegally obtained funds as legitimate income.
AML initiatives are crucial in maintaining the integrity of financial systems. They help in detecting and preventing activities such as fraud, corruption, and terrorist financing.
The significance of AML efforts can be summarised in several key points:
- Protects economies from financial crime-related instability
- Enhances public trust in financial institutions
- Ensures compliance with international regulations
By integrating robust AML strategies, financial organisations can prevent potential threats and mitigate risks. These strategies help them contribute to a more secure and transparent financial environment.
The Evolution of AML Investigation Tools
The landscape of AML investigation tools has evolved significantly over the years. Initially, manual processes and simple databases were the norm for tracking illicit activities. These rudimentary tools, while effective at their time, had limitations in handling complex financial crimes.
As financial crime grew more sophisticated, so did the technology to combat it. Today's financial environment demands advanced tools capable of real-time analysis and threat detection. Modern AML tools leverage technologies like artificial intelligence and machine learning, making them indispensable in identifying and mitigating risks efficiently.
Key Types of AML Investigation Tools
AML investigation tools come in various forms, each serving a unique purpose in combating money laundering. Among them, transaction monitoring systems help identify unusual behaviour, flagging activities that deviate from normal patterns. Name screening tools are also vital, allowing institutions to check clients against global sanction lists.
In the current technological landscape, AI and machine learning have revolutionised these tools. These technologies provide the capability to process vast amounts of data quickly, pinpointing anomalies with higher accuracy than ever before. Machine learning models enhance the ability of tools to adapt to new and evolving patterns of financial crime.
Moreover, some tools focus on customer due diligence, ensuring thorough KYC (Know Your Customer) protocols are observed. Here’s a list of key AML tools:
- Transaction Monitoring Systems
- Name Screening Solutions
- Customer Due Diligence Platforms
- AI and Machine Learning-Driven Systems
Each tool is integral to a comprehensive AML strategy, enabling better detection and prevention of financial crimes.
{{cta-first}}
Automating Detection with AML Investigation Software
Automation in AML investigation software is a game-changer. It helps identify suspicious activities by consistently scanning transactions for irregular patterns. Automated systems reduce the manual workload, allowing investigators to focus on more complex cases.
Integration with existing financial systems is crucial for seamless operations. AML software can interface with these systems to provide real-time alerts, making response actions quicker. This connectivity ensures that financial institutions maintain a strong stance against money laundering, as automated alerts allow for swift intervention. Consequently, it enhances the overall efficiency and reliability of AML processes.
Risk Assessment Tools: Gauging Transaction and Customer Risks
Advanced risk assessment tools play a vital role in financial crime prevention. These tools evaluate potential risks associated with both transactions and customers. By analysing various risk factors, they help financial institutions categorise and prioritise cases for further investigation.
Real-time monitoring is paramount in risk assessment. It allows institutions to quickly identify and address potential threats as they arise. This proactive approach not only prevents illicit activities but also ensures compliance with regulatory standards. Real-time capabilities empower financial entities to react promptly, safeguarding them against evolving threats in the financial landscape.
Regulatory Compliance and AML Tools
AML tools are essential in navigating the complex landscape of regulatory requirements. They help organisations to automatically ensure that their practices align with regional and international anti-money laundering laws. By leveraging these tools, compliance teams can stay updated on regulatory changes and implement necessary adjustments quickly.
Many organisations have successfully used AML tools to maintain compliance with evolving standards. For instance, financial institutions have reduced regulatory penalties by implementing comprehensive software solutions. These case studies emphasise the importance of technology in safeguarding institutions against legal risks, showcasing how strategic use of AML tools leads to successful compliance outcomes.
The Future of AML Technology: Trends to Watch
The landscape of AML technology is set to change significantly over the coming years. One major trend is the increased use of artificial intelligence to enhance the accuracy of threat detection. This technology promises to refine AML processes by reducing false positives and predicting suspicious activities with greater precision.
Another critical area is the monitoring of blockchain and cryptocurrencies. As digital currencies become more mainstream, AML tools must adapt to address the risks posed by these technologies. This involves developing capabilities to track and analyse cryptocurrency transactions, thus ensuring compliance with evolving regulatory frameworks. Organisations need to prepare for these changes to stay ahead in the fight against financial crime.
Selecting the Right AML Investigation Tools for Your Organisation
Choosing the appropriate AML investigation tools is a crucial decision for organizations. The right software should align with the specific needs and complexities of your operations. Careful evaluation ensures these tools provide meaningful insights and ease regulatory compliance processes.
Here are key criteria for selecting AML tools:
- Scalability: Ensure the tool can grow with your organisation.
- Customisation: Tailor the features to fit organisational needs.
- User-friendliness: Ease of use is vital for efficiency.
- Integration: Compatibility with existing systems is crucial.
- Support and training: Access to continuous support enhances tool effectiveness.
Scalability and customisation remain paramount in this selection process. These features ensure the tool adapts to changing regulatory landscapes and evolving business models. With scalable and customisable options, organisations can effectively manage risks and maintain robust compliance.
{{cta-whitepaper}}
Overcoming Challenges and Maximising the Benefits of AML Tools
Financial crime investigators often encounter significant challenges when using AML tools. Common issues include managing false positives and integrating systems seamlessly with existing workflows. These hurdles can affect the efficiency of the investigation process.
To maximise the benefits, it's crucial to implement strategies that enhance accuracy and streamline operations. Leveraging machine learning and AI can significantly reduce false positives, providing investigators with more relevant alerts. Furthermore, ongoing training and support for users ensure the tools are utilised to their full potential. This optimises the efficacy of AML tools and reinforces the overall anti-money laundering efforts.
Conclusion: Staying Ahead in the Fight Against Financial Crime
In conclusion, embracing Tookitaki's FinCense for fraud investigation can dramatically enhance the efficiency and effectiveness of your AML processes. With its Case Manager feature, investigations and reporting are simplified, enabling a unified workflow that centralises alert investigation across FRAML, Smart Screening, and Dynamic Risk Scoring within a single case.
The Single Window Investigation capability allows teams to access all relevant information—customer details, transaction history, alert records, and linked accounts—on one screen, making it easier to investigate and dispose of cases quickly and accurately.
Additionally, automated report generation ensures compliance with local regulations while providing operational-level insights to monitor system performance and team productivity. Workflow automation smartly manages low-risk alerts, merges cases, and optimises allocations, allowing investigators to focus on higher-priority tasks.
Finally, the dynamic dashboard facilitates agile and decentralised team operations, offering complete visibility with real-time updates on alerts and case lifecycles at a macro level. By leveraging Tookitaki's FinCense, financial crime investigators can not only streamline their processes but also stay ahead in the ongoing fight against fraud.
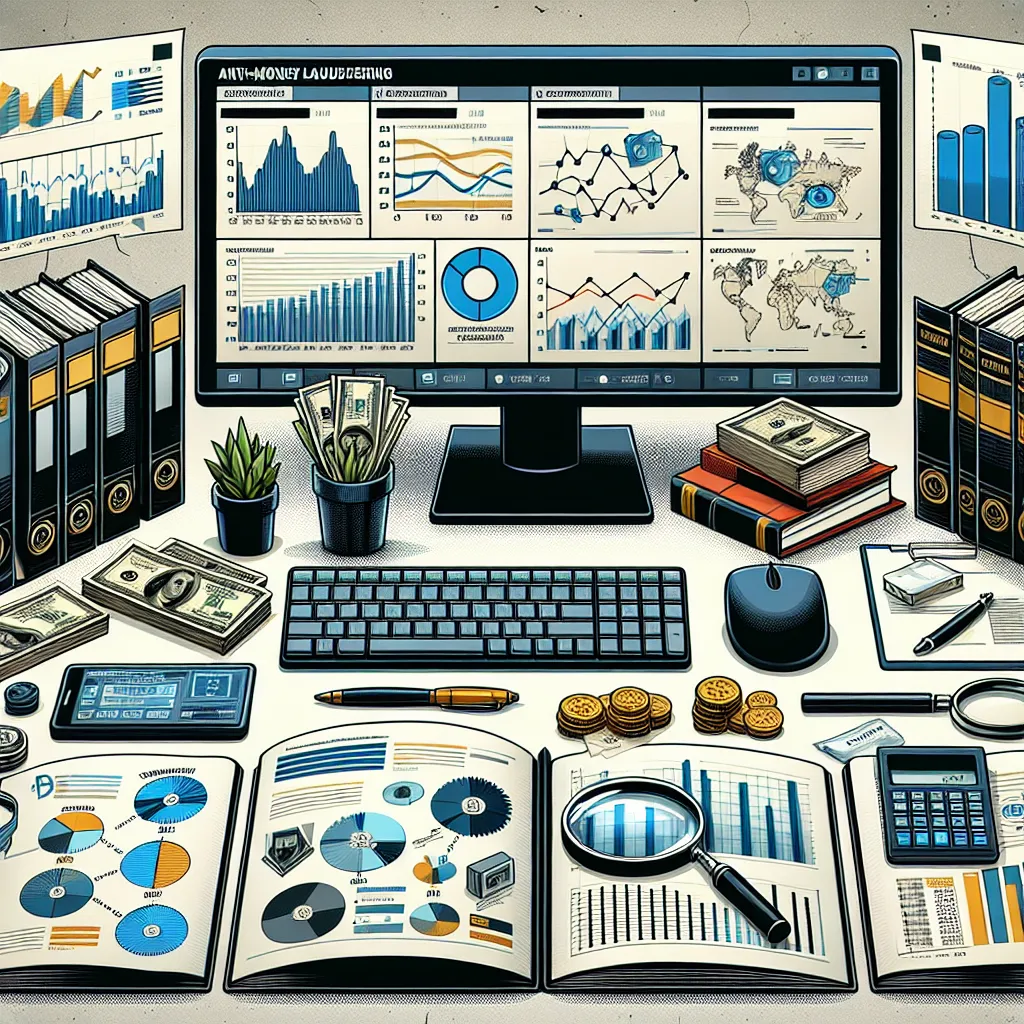
AML Transaction Monitoring Software Explained: Key Features & Best Practices
AML transaction monitoring software has become a mission-critical tool for modern financial institutions.
As financial crime grows more complex and regulators raise the bar on compliance, static or outdated systems are no longer enough. Today’s banks and fintechs need intelligent, adaptive software that not only flags suspicious activity but also learns, evolves, and reduces false positives over time. In this blog, we explore how next-generation AML transaction monitoring software works, the key features to look for, and how to future-proof your compliance operations in an increasingly dynamic threat landscape.
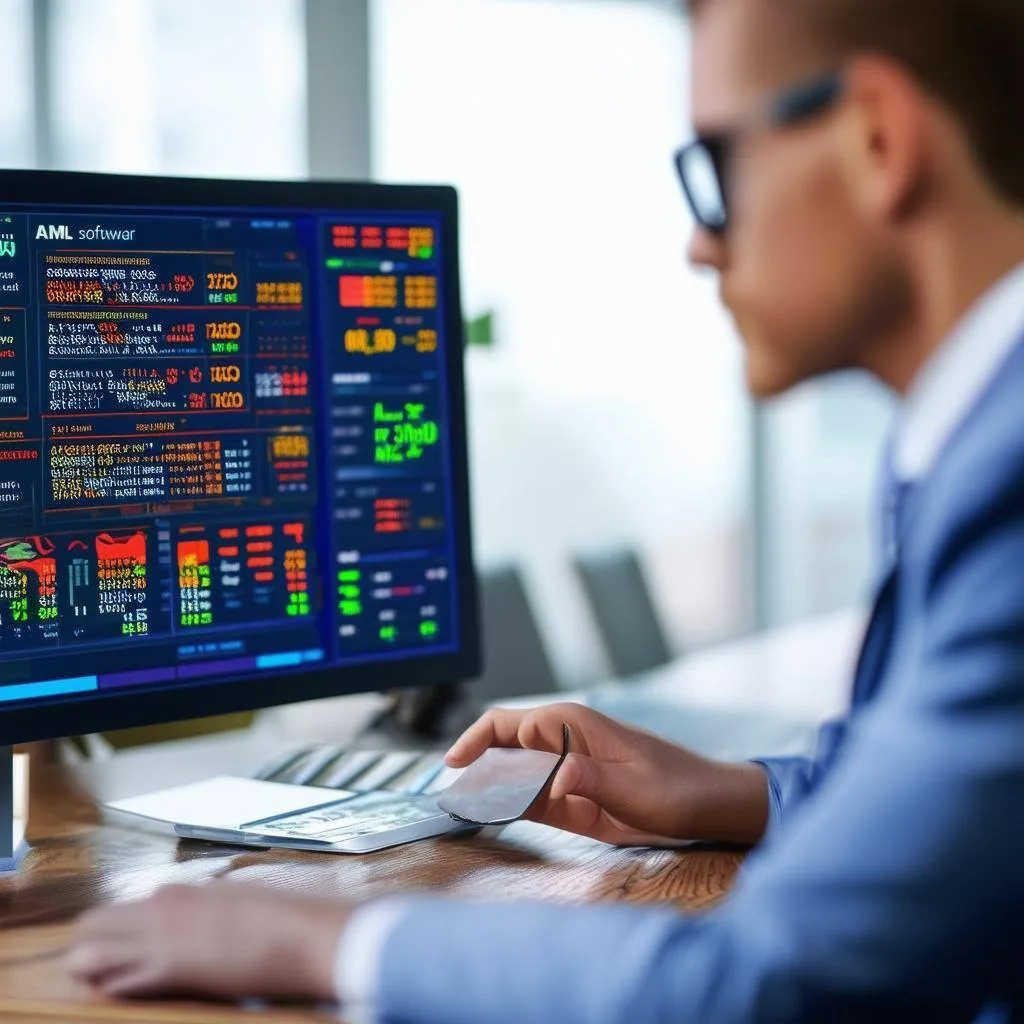
Understanding AML Transaction Monitoring Software
AML Transaction Monitoring Software is a critical tool for financial institutions, enabling them to detect and prevent illicit financial activities such as money laundering, fraud, and terrorism financing. By continuously analyzing transactional data, these systems identify unusual patterns, anomalies, and red flags that could indicate financial crime.
How Does AML Transaction Monitoring Software Work?
🔹 Real-Time Data Analysis: Advanced algorithms scan transaction data from multiple sources, instantly flagging suspicious activities. This proactive monitoring ensures risks are identified before they escalate.
🔹 Regulatory Compliance: Compliance with global AML regulations is non-negotiable. AML Transaction Monitoring Software helps financial institutions stay compliant, mitigating the risk of hefty fines and reputational damage.
🔹 AI & Machine Learning Enhancements: Traditional rule-based monitoring often leads to high false positives. AI-driven systems adapt and evolve, reducing false alarms and focusing on genuine threats with precision.
🔹 Instant Alerts & Response: The ability to detect and respond in real-time is a game-changer. Timely alerts allow compliance teams to act swiftly, preventing financial criminals from exploiting gaps in the system.
As financial crime tactics grow more sophisticated, the role of AML Transaction Monitoring Software becomes even more vital. Financial institutions that leverage AI-powered, real-time monitoring systems can stay ahead of threats while ensuring seamless compliance.
{{cta-first}}
The Importance of AML Transaction Monitoring in Financial Crime Prevention
For financial institutions, AML Transaction Monitoring Software is more than just a compliance requirement—it’s a strategic defence against financial crime. Regulatory non-compliance can lead to crippling fines and reputational damage, making robust monitoring systems essential.
Why AML Transaction Monitoring Software is Critical
🔹 Regulatory Compliance & Risk Mitigation – Global AML regulations demand continuous monitoring of transactions. A well-implemented AML Transaction Monitoring Software ensures financial institutions meet compliance requirements while minimizing legal and reputational risks.
🔹 Proven Impact on Financial Crime Prevention – Case studies show that banks leveraging AI-powered transaction monitoring solutions report a significant drop in suspicious activity. The right software enhances detection, reduces false positives, and prevents illicit transactions before they cause harm.
🔹 Seamless Integration with Other Crime Prevention Tools – Transaction monitoring doesn’t operate in a vacuum. When integrated with AI-driven fraud detection, name screening, and behavioural analytics, it creates a multi-layered defence system against evolving threats.
🔹 Big Data & AI for Smarter Detection – The ability to analyze massive data sets in real-time allows institutions to uncover hidden patterns linked to money laundering and fraud. AI and machine learning-driven AML Transaction Monitoring Software fine-tune risk detection, enabling institutions to detect sophisticated threats with greater accuracy.
With financial criminals constantly evolving their tactics, institutions that invest in advanced AML Transaction Monitoring Software stay ahead of threats, ensuring both compliance and security in an increasingly complex financial landscape.
Challenges and Solutions in Transaction Monitoring
Despite its importance, AML Transaction Monitoring Software comes with challenges that financial institutions must address to ensure accurate threat detection and compliance efficiency. From high false positives to evolving financial crime tactics, tackling these issues requires a mix of technology, fine-tuning, and expert oversight.
Key Challenges & How to Overcome Them
🔹 High Volume & Complexity of Transactions
Financial institutions process millions of transactions daily, making it difficult to distinguish between legitimate activity and illicit patterns. Solution: AI-driven AML Transaction Monitoring Software can analyze massive datasets in real-time, filtering out noise while identifying genuine threats.
🔹 False Positives Overload
Excessive false positives waste compliance resources and slow down investigations. Solution: Advanced AI/ML models continuously refine detection parameters, reducing false alerts and ensuring investigators focus on high-risk activities.
🔹 Calibrating Risk Thresholds & Parameters
Setting monitoring baselines is complex—too strict, and you trigger unnecessary alerts; too lenient, and risks go undetected. Solution: Risk-based approaches powered by behavioural analytics allow institutions to dynamically adjust thresholds based on customer profiles and transactional history.
🔹 Human Expertise & Alert Interpretation
Even the most advanced systems require human oversight. Solution: Skilled analysts are essential to differentiate between actual threats and benign anomalies. Continuous training and AI-assisted decision-making tools can enhance efficiency.
🔹 Evolving Money Laundering Tactics
Financial criminals constantly adapt to bypass detection. Solution: AML Transaction Monitoring Software must evolve too. Regular updates, AI-driven anomaly detection, and leveraging shared intelligence from compliance networks keep systems one step ahead.
The Future of AML Transaction Monitoring
As financial criminals become more sophisticated, the need for intelligent, adaptive AML Transaction Monitoring Software has never been greater. Institutions that leverage AI, machine learning, and collaborative intelligence will be best positioned to mitigate risk, improve detection accuracy, and enhance compliance effectiveness.
Key Features of AML Transaction Monitoring Systems
To effectively combat financial crime, AML Transaction Monitoring Software must be equipped with advanced capabilities that enhance detection accuracy, streamline compliance, and reduce operational inefficiencies.
Essential Features of AML Transaction Monitoring Software
🔹 Real-Time Transaction Monitoring
Instant detection of suspicious transactions is critical in preventing financial crime. AI-powered AML Transaction Monitoring Software analyzes transactions as they happen, allowing compliance teams to respond swiftly before illicit activity escalates.
🔹 Advanced Data Analytics & Pattern Recognition
With financial criminals using increasingly sophisticated laundering techniques, modern AML software leverages big data, machine learning, and behavioural analytics to detect hidden patterns and anomalies that rule-based systems might miss.
🔹 Risk-Based Customer Due Diligence (CDD & KYC Integration)
A strong AML framework starts with understanding customer behaviour. AML Transaction Monitoring Software integrates with Know Your Customer (KYC) and risk assessment systems to flag high-risk entities and enhance due diligence.
🔹 Adaptive Risk Scoring & Threshold Calibration
Static rules lead to excessive false positives or missed risks. AI-driven calibration dynamically adjusts risk thresholds based on transaction behaviour, ensuring accurate threat detection without overwhelming compliance teams.
🔹 Automated Alerts & Case Management
Efficiency is key. The best AML software provides automated alerts, case prioritization, and workflow automation, helping compliance teams focus on high-risk transactions and streamline investigations.
Why These Features Matter
In today’s fast-moving financial crime landscape, financial institutions need agile, intelligent, and scalable AML Transaction Monitoring Software to stay ahead. Leveraging these advanced features ensures stronger compliance, better fraud detection, and reduced operational burden.
Best Practices for Implementing AML Transaction Monitoring Software
Implementing AML Transaction Monitoring Software effectively requires a strategic approach that aligns with an institution’s risk profile, compliance obligations, and operational needs. Here’s how to maximize its impact:
Key Best Practices for AML Transaction Monitoring Implementation
🔹 Strengthen Customer Due Diligence (CDD)
A risk-based approach is essential for effective transaction monitoring. By analyzing customer behaviour, transaction history, and risk profiles, institutions can set precise monitoring thresholds, making it easier to detect anomalies without excessive false alerts.
🔹 Customize Software for Institutional Needs
A one-size-fits-all approach doesn’t work in AML compliance. Every financial institution has unique risks—from cross-border transactions to high-risk customer segments. AML Transaction Monitoring Software should be customizable to align with the organization’s specific risk landscape.
🔹 Train Compliance Teams Regularly
Even the most sophisticated AML software requires skilled analysts to interpret alerts and refine detection models. Continuous training ensures compliance teams stay updated on new system features, emerging financial crime tactics, and regulatory changes.
🔹 Regular System Reviews & Optimization
Financial criminals constantly evolve their tactics—so should your AML Transaction Monitoring Software. Periodic reviews, data quality assessments, and AI model recalibration help enhance detection accuracy and adapt to new threats.
Why These Best Practices Matter
By integrating these best practices, financial institutions can maximize the efficiency of their AML Transaction Monitoring Software, ensuring stronger compliance, reduced false positives, and faster response to suspicious activities.
The Future of AML Transaction Monitoring Software
As financial crime tactics grow more sophisticated, the future of AML Transaction Monitoring Software lies in predictive intelligence, regulatory adaptability, and next-gen technology. Institutions that embrace these innovations will be better equipped to prevent financial crime before it happens.
Emerging Trends in AML Transaction Monitoring Software
🔹 Predictive Analytics for Proactive Crime Prevention
The future of AML monitoring is anticipatory, not just reactive. AI-driven predictive analytics can forecast potential money laundering risks before they materialize, allowing institutions to intervene earlier and strengthen fraud prevention strategies.
🔹 Data Privacy & Protection in a Digital-First Era
With increasing global regulations on data security (e.g., GDPR, PDPA, CCPA), financial institutions must ensure AML Transaction Monitoring Software aligns with strict data protection standards. Advanced encryption, secure storage, and AI-driven anomaly detection will play a key role in securing transaction data.
🔹 Cross-Border Compliance & Regulatory Adaptability
Transaction monitoring for cross-border payments is becoming more complex due to varied AML regulations across jurisdictions. Next-gen software must integrate multi-regional compliance frameworks and AI-driven risk scoring to adapt seamlessly to different regulatory landscapes.
🔹 Blockchain & Digital Asset Monitoring
Blockchain’s transparency and immutability offer promising solutions for AML. Future AML Transaction Monitoring Software will leverage blockchain-powered monitoring to track illicit transactions across decentralized networks, improving traceability in cryptocurrency and digital asset compliance.
The Road Ahead
As financial criminals evolve, AML Transaction Monitoring Software must become faster, smarter, and more adaptive. The integration of AI, predictive analytics, blockchain, and global compliance intelligence will redefine the future of financial crime prevention.
{{cta-whitepaper}}
Conclusion: Future-Proof Your AML Compliance with FinCense
Financial crime is evolving, and compliance demands efficiency, accuracy, and scalability. Tookitaki’s FinCense delivers next-gen AML Transaction Monitoring Software, ensuring 100% risk coverage, 90% detection accuracy, and 50% lower compliance costs.
Why FinCense?
✔️ Real-time AML Transaction Monitoring – Detect and mitigate risks instantly.
✔️ AI-Powered Smart Screening & Risk Scoring – Reduce false positives by up to 90%.
✔️ Automated Case Management – Investigate customers, not just alerts, with 40% faster handling.
✔️ Seamless KYC & Onboarding Integration – Instant, risk-based customer profiling.
With FinCense, compliance teams stay ahead of threats, ensuring faster, smarter, and cost-effective AML compliance.
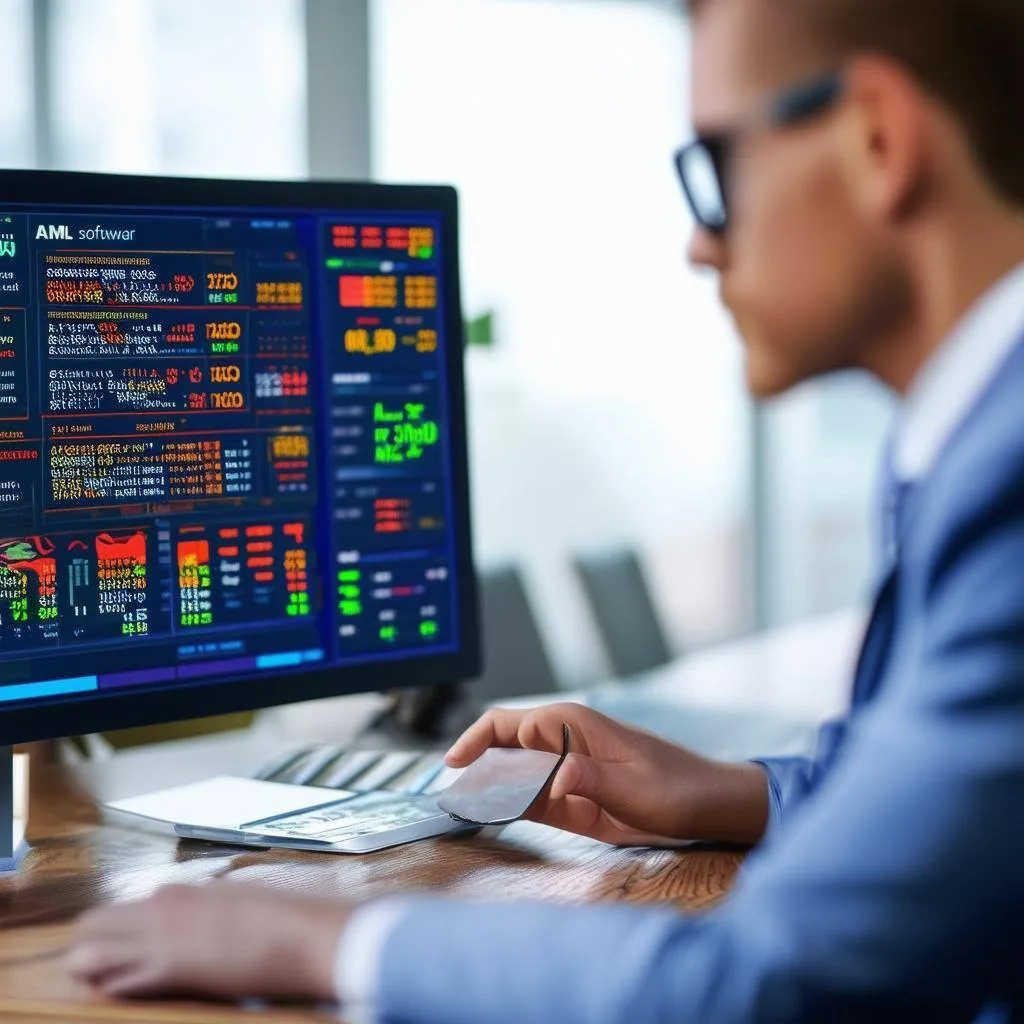
AML Fraud Detection: The Hidden Threats Banks Miss in 2025
Financial institutions worldwide face a massive challenge as criminals launder an estimated $2 trillion annually through banks. Banks pour resources into compliance programs but still miss key threats. This failure has resulted in $342 billion worth of AML fines since 2019.
The digital world of financial crime changes rapidly. Regulators have already issued 80 AML fines worth $263 million in the first half of 2024. These numbers show a 31% jump from 2023's figures. Criminals actively exploit the gaps created by banks' separate approaches to AML and fraud detection.
Banks need to understand the hidden threats they might miss in 2025. Traditional systems often fail to catch sophisticated schemes. A more integrated approach could help financial institutions protect themselves better against new risks.

The Evolution of Money Laundering Techniques in 2025
Criminal organizations keep finding new ways to commit financial crimes. Their money laundering techniques have become more sophisticated in 2025. These criminals now use complex technology-based strategies because law enforcement targets conventional methods.
Traditional vs. modern laundering methods
Money launderers used to rely on cash-heavy businesses, physical assets, and offshore accounts. Today's criminals prefer digital methods that give them better anonymity and speed. The International Monetary Fund reports that money laundering makes up about 5% of the global GDP. These numbers show how massive this criminal enterprise has become.
Modern criminals now infiltrate legitimate businesses and use complex corporate structures across borders. German authorities reported their highest financial crime damage from organized groups in 10 years during 2023. This surge proves how effective these new methods have become.
{{cta-first}}
The rise of synthetic identity fraud
Synthetic identity fraud combines real and fake information to create "Frankenstein IDs" that look genuine. This crime has become the fastest-growing financial fraud in the United States. Banks lose an estimated PHP 353.63 billion to this scheme. Each fraudulent account costs about PHP 884,063.70 on average.
These fake identities target the most vulnerable people. Criminals use children's Social Security numbers 51 times more often than others. They also target elderly and homeless people who rarely check their credit reports.
Crypto-mixing and cross-chain transactions
Cross-chain crime leads the way in cryptocurrency laundering. This technique, also called "chain-hopping," swaps cryptocurrencies between different tokens or blockchains quickly to hide their criminal sources.
Criminals have laundered PHP 412.56 billion worth of illegal crypto through cross-chain services. They prefer privacy-focused bridges like Thorchain and Incognito that use zero-knowledge proofs to hide transaction details. RenBridge alone has helped launder at least PHP 31.83 billion in criminal proceeds.
AI-powered laundering schemes
AI has changed how criminals launder money. They now use AI algorithms to create realistic fake identities, automate complex transactions, and generate convincing business documents to make illegal money look legal.
AI helps create synthetic identities for financial crimes and bypass traditional verification methods. Criminals value this technology because it automates "structured" transactions. They split large amounts into smaller transfers across multiple accounts to avoid detection systems.
Why Traditional AML Systems Fail to Detect New Threats
Banks invest heavily in compliance but still struggle to catch sophisticated money laundering schemes. Their existing systems can't keep up with new criminal tactics. This creates dangerous blind spots that lead to billions in fines.
Rule-based limitations in complex scenarios
AML systems today depend too much on fixed rules and thresholds that criminals know how to bypass. These rigid systems flood analysts with false alarms, which makes real threats harder to spot. A Chief AML Officer at a financial institution learned they could turn off several detection rules without affecting the number of suspicious activity reports.
Rule-based monitoring has a basic flaw - it can't place transactions in context. The system doesn't know the difference between a pizza delivery worker getting drug money from another state and a student receiving help from family. This makes investigators tune out alerts and miss actual suspicious activity.
Data silos preventing holistic detection
Teams that don't share information make it harder to catch financial crimes. Research shows 55% of companies work in silos, and 54% of financial leaders say this blocks progress. The cost is staggering - Fortune 500 companies lose PHP 1856.53 billion each year by not sharing knowledge between teams.
The Danske Bank scandal shows what can go wrong. The bank couldn't combine its Estonian branch's systems with main operations, which left a gap where suspicious transactions went unnoticed for years. Important data stuck in separate systems or departments makes compliance work slow and prone to mistakes.
Outdated risk assessment models
Most banks still use basic customer risk profiles that quickly become stale. They collect information when accounts open but rarely update it. Banks expect customers to refresh their own details, which almost never happens.
Old-style risk tools built on spreadsheets and static reports can't handle large-scale data analysis. This limits their ability to spot patterns that could paint a better risk picture. Many banks only check risk once a year - a process that drags on for months. Criminals exploit this gap between their new methods and the bank's outdated models.
Hidden Threats Banks Are Missing Today
Financial institutions can't keep up with evolving money laundering tactics that exploit gaps between traditional AML and fraud detection systems. Criminals move billions undetected by using sophisticated threats that operate in detection blind spots.
Smurfing 2.0: Micro-transactions across multiple platforms
Traditional "smurfing" has grown beyond breaking large transactions into smaller ones. Criminals now spread tiny amounts across many digital channels in what experts call "micro-money laundering." They avoid suspicion by making hundreds of small transactions that look legitimate on their own.
This approach works well because:
- Digital payment platforms enable quick, high-volume, small-value transactions
- Alert systems miss these micro-transfers since they stay below reporting limits
- Spreading transactions across platforms prevents banks from seeing the full picture
Legitimate business infiltration
Criminal networks in the EU have found a new way to hide their activities - 86% now use legal business structures as cover. Cash-heavy businesses make perfect fronts for laundering money and create unfair advantages that hurt honest companies.
Criminals naturally blend legal and illegal operations through high-level infiltration or direct ownership. Some companies exist purely as fronts for criminal activities, while bad actors buy others to achieve their long-term criminal goals.
Real-time payment exploitation
Real-time payments give fraudsters the perfect chance to strike. These transactions can't be reversed once started, which leaves banks no time to step in. Fraud losses jumped 164% in just two years after real-time payment services launched in the US and UK.
Banks struggle to keep pace with these systems that process transactions around the clock. The risk grows since delayed detection means criminals have already moved the money before anyone spots the fraud patterns.
Mule account networks
Modern money laundering operations rely heavily on sophisticated mule networks. Between January 2022 and September 2023, just 25 banks removed 194,084 money mules from their systems. The National Fraud Database only received reports for 37% of these accounts.
Mule handlers recruit people to move dirty money through personal accounts. This creates complex patterns that hide the money's true path. Many banks still can't detect customers who knowingly join these schemes, especially when transactions appear normal on the surface.
AML vs Fraud Detection: Bridging the Critical Gap
Financial institutions have managed to keep separate teams to fight fraud and money laundering. This setup creates dangerous gaps in their defensive armor. Criminal operations now blur the lines between fraud and laundering activities, which makes us think about these long-standing divisions.
Understanding the fundamental differences
AML and fraud detection work differently within financial institutions. Chief Compliance Officers watch over AML as a compliance-driven operation. Meanwhile, Chief Risk Officers handle fraud detection as a risk management function. The main difference shows in their focus. AML stops criminals from making illegal money look legitimate. Fraud prevention protects customers and institutions from losing money.
Their approaches work quite differently:
- Fraud monitoring uses live detection to stop fraud before it hits customers
- AML monitoring looks at detailed data analysis to spot suspicious patterns and meet legal requirements
Where traditional approaches create blind spots
Separate teams create major weak points in the system. Money laundering usually follows fraud, but most institutions look at these risks separately. This separation leads to:
- Teams doing the same alert reviews and case investigations twice
- Risk assessment models that can't see connected activities
- Resources, systems and data management that don't work well together
Separate approaches miss a key point: fraudulent transactions often point to money laundering activity. This needs suspicious activity reports even without clear connections.
The FRAML approach: Integrated protection
FRAML (Fraud Risk Assessment and Management Lifecycle) brings together fraud management and AML principles into one framework. This integrated way shows that these financial crimes share common patterns and risk factors.
The benefits show up quickly:
- Risk assessments that look at both fraud and money laundering threats
- Teams share data analytics and investigations to spot suspicious transactions faster
- Companies can save 20-30% through better systems and processes
Case study: How integration caught what siloed systems missed
A prominent North American Tier 1 bank tried a FRAML analytics approach. They fed data from multiple sources into one accessible interface. These sources included fraud detection, KYC, documentation, sanctions, and transaction monitoring. This change helped them catch 30% more mule accounts in just one year.
A mid-tier payments startup saw similar results. They improved their work output by 20% after bringing fraud and AML detection together. Their team projects that this number could reach 40% over the next year.
{{cta-whitepaper}}
Conclusion
Criminal money laundering methods have evolved beyond what traditional detection systems can handle. Banks that keep their AML and fraud detection systems separate create weak spots that criminals actively target.
Banks need complete solutions to connect fraud prevention with AML compliance. The FRAML approach works well - early users have seen their threat detection improve by 30%. Tookitaki's AFC Ecosystem and FinCense platform deliver this integrated protection. They merge up-to-the-minute intelligence sharing with complete compliance features.
Financial institutions can now better shield themselves from new threats like synthetic identity fraud, crypto-mixing, and complex mule account networks. Both large banks and payment startups have proven the worth of unified systems. Their success stories show better detection rates and budget-friendly results through optimized operations.
The battle against financial crime demands continuous adaptation and alertness. Traditional methods are not enough as criminals keep improving their tactics. Banks must accept new ideas that combine advanced analytics, live monitoring, and community-driven intelligence to remain competitive against evolving threats in 2025 and beyond.

Beyond Alerts: How AML Investigation Tools Are Transforming Compliance Operations
Financial crime is evolving—and your investigation tools should too.
As regulatory pressure intensifies and criminal tactics grow more sophisticated, the ability to investigate money laundering efficiently has become mission-critical for financial institutions. Traditional approaches often fall short—burdened by fragmented data, alert fatigue, and manual processes.
In this blog, we explore how modern AML investigation tools empower compliance teams with smarter workflows, real-time insights, and collaborative intelligence. From case management to behavioural analytics, we’ll unpack the features that matter most—and how to choose the right tools for your institution.
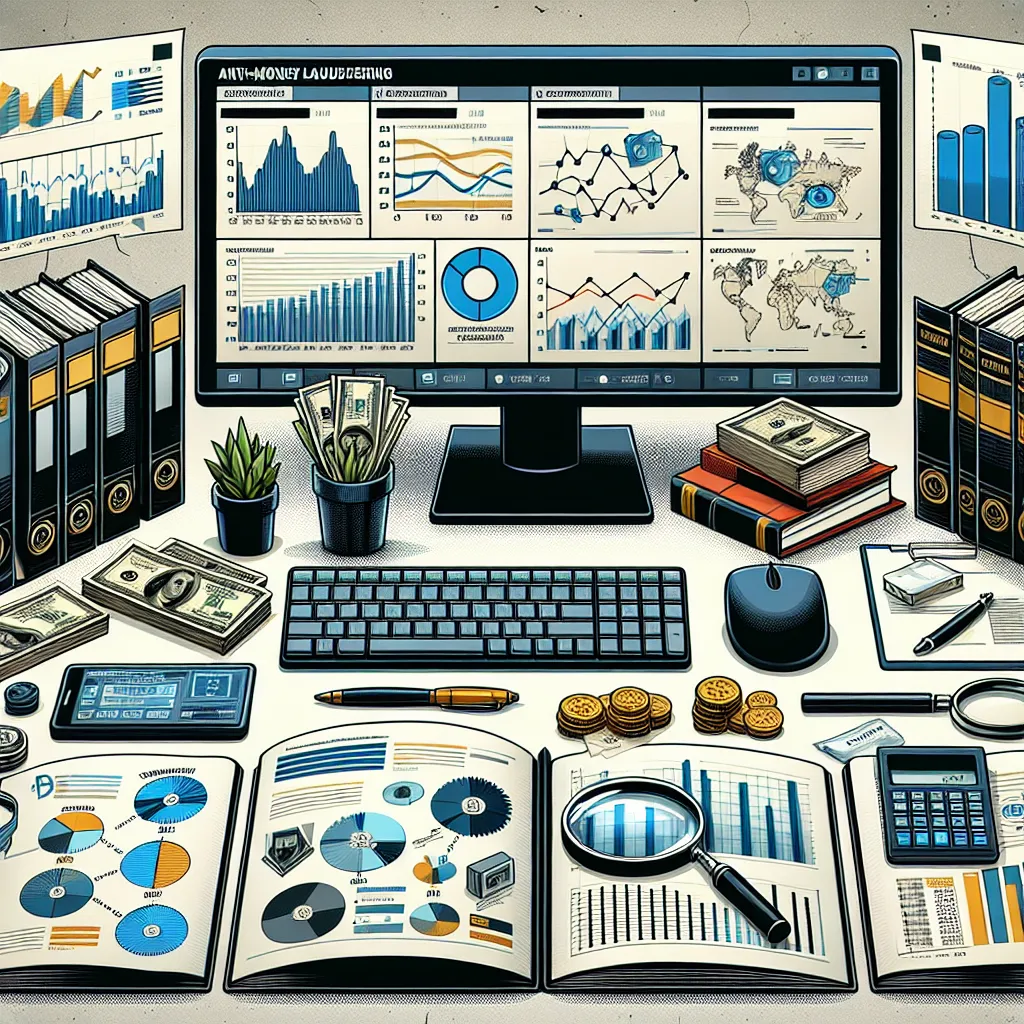
Understanding AML and Its Significance in Financial Crime Prevention
Anti-Money Laundering, commonly known as AML, refers to a set of laws and procedures. These are designed to prevent criminals from disguising illegally obtained funds as legitimate income.
AML initiatives are crucial in maintaining the integrity of financial systems. They help in detecting and preventing activities such as fraud, corruption, and terrorist financing.
The significance of AML efforts can be summarised in several key points:
- Protects economies from financial crime-related instability
- Enhances public trust in financial institutions
- Ensures compliance with international regulations
By integrating robust AML strategies, financial organisations can prevent potential threats and mitigate risks. These strategies help them contribute to a more secure and transparent financial environment.
The Evolution of AML Investigation Tools
The landscape of AML investigation tools has evolved significantly over the years. Initially, manual processes and simple databases were the norm for tracking illicit activities. These rudimentary tools, while effective at their time, had limitations in handling complex financial crimes.
As financial crime grew more sophisticated, so did the technology to combat it. Today's financial environment demands advanced tools capable of real-time analysis and threat detection. Modern AML tools leverage technologies like artificial intelligence and machine learning, making them indispensable in identifying and mitigating risks efficiently.
Key Types of AML Investigation Tools
AML investigation tools come in various forms, each serving a unique purpose in combating money laundering. Among them, transaction monitoring systems help identify unusual behaviour, flagging activities that deviate from normal patterns. Name screening tools are also vital, allowing institutions to check clients against global sanction lists.
In the current technological landscape, AI and machine learning have revolutionised these tools. These technologies provide the capability to process vast amounts of data quickly, pinpointing anomalies with higher accuracy than ever before. Machine learning models enhance the ability of tools to adapt to new and evolving patterns of financial crime.
Moreover, some tools focus on customer due diligence, ensuring thorough KYC (Know Your Customer) protocols are observed. Here’s a list of key AML tools:
- Transaction Monitoring Systems
- Name Screening Solutions
- Customer Due Diligence Platforms
- AI and Machine Learning-Driven Systems
Each tool is integral to a comprehensive AML strategy, enabling better detection and prevention of financial crimes.
{{cta-first}}
Automating Detection with AML Investigation Software
Automation in AML investigation software is a game-changer. It helps identify suspicious activities by consistently scanning transactions for irregular patterns. Automated systems reduce the manual workload, allowing investigators to focus on more complex cases.
Integration with existing financial systems is crucial for seamless operations. AML software can interface with these systems to provide real-time alerts, making response actions quicker. This connectivity ensures that financial institutions maintain a strong stance against money laundering, as automated alerts allow for swift intervention. Consequently, it enhances the overall efficiency and reliability of AML processes.
Risk Assessment Tools: Gauging Transaction and Customer Risks
Advanced risk assessment tools play a vital role in financial crime prevention. These tools evaluate potential risks associated with both transactions and customers. By analysing various risk factors, they help financial institutions categorise and prioritise cases for further investigation.
Real-time monitoring is paramount in risk assessment. It allows institutions to quickly identify and address potential threats as they arise. This proactive approach not only prevents illicit activities but also ensures compliance with regulatory standards. Real-time capabilities empower financial entities to react promptly, safeguarding them against evolving threats in the financial landscape.
Regulatory Compliance and AML Tools
AML tools are essential in navigating the complex landscape of regulatory requirements. They help organisations to automatically ensure that their practices align with regional and international anti-money laundering laws. By leveraging these tools, compliance teams can stay updated on regulatory changes and implement necessary adjustments quickly.
Many organisations have successfully used AML tools to maintain compliance with evolving standards. For instance, financial institutions have reduced regulatory penalties by implementing comprehensive software solutions. These case studies emphasise the importance of technology in safeguarding institutions against legal risks, showcasing how strategic use of AML tools leads to successful compliance outcomes.
The Future of AML Technology: Trends to Watch
The landscape of AML technology is set to change significantly over the coming years. One major trend is the increased use of artificial intelligence to enhance the accuracy of threat detection. This technology promises to refine AML processes by reducing false positives and predicting suspicious activities with greater precision.
Another critical area is the monitoring of blockchain and cryptocurrencies. As digital currencies become more mainstream, AML tools must adapt to address the risks posed by these technologies. This involves developing capabilities to track and analyse cryptocurrency transactions, thus ensuring compliance with evolving regulatory frameworks. Organisations need to prepare for these changes to stay ahead in the fight against financial crime.
Selecting the Right AML Investigation Tools for Your Organisation
Choosing the appropriate AML investigation tools is a crucial decision for organizations. The right software should align with the specific needs and complexities of your operations. Careful evaluation ensures these tools provide meaningful insights and ease regulatory compliance processes.
Here are key criteria for selecting AML tools:
- Scalability: Ensure the tool can grow with your organisation.
- Customisation: Tailor the features to fit organisational needs.
- User-friendliness: Ease of use is vital for efficiency.
- Integration: Compatibility with existing systems is crucial.
- Support and training: Access to continuous support enhances tool effectiveness.
Scalability and customisation remain paramount in this selection process. These features ensure the tool adapts to changing regulatory landscapes and evolving business models. With scalable and customisable options, organisations can effectively manage risks and maintain robust compliance.
{{cta-whitepaper}}
Overcoming Challenges and Maximising the Benefits of AML Tools
Financial crime investigators often encounter significant challenges when using AML tools. Common issues include managing false positives and integrating systems seamlessly with existing workflows. These hurdles can affect the efficiency of the investigation process.
To maximise the benefits, it's crucial to implement strategies that enhance accuracy and streamline operations. Leveraging machine learning and AI can significantly reduce false positives, providing investigators with more relevant alerts. Furthermore, ongoing training and support for users ensure the tools are utilised to their full potential. This optimises the efficacy of AML tools and reinforces the overall anti-money laundering efforts.
Conclusion: Staying Ahead in the Fight Against Financial Crime
In conclusion, embracing Tookitaki's FinCense for fraud investigation can dramatically enhance the efficiency and effectiveness of your AML processes. With its Case Manager feature, investigations and reporting are simplified, enabling a unified workflow that centralises alert investigation across FRAML, Smart Screening, and Dynamic Risk Scoring within a single case.
The Single Window Investigation capability allows teams to access all relevant information—customer details, transaction history, alert records, and linked accounts—on one screen, making it easier to investigate and dispose of cases quickly and accurately.
Additionally, automated report generation ensures compliance with local regulations while providing operational-level insights to monitor system performance and team productivity. Workflow automation smartly manages low-risk alerts, merges cases, and optimises allocations, allowing investigators to focus on higher-priority tasks.
Finally, the dynamic dashboard facilitates agile and decentralised team operations, offering complete visibility with real-time updates on alerts and case lifecycles at a macro level. By leveraging Tookitaki's FinCense, financial crime investigators can not only streamline their processes but also stay ahead in the ongoing fight against fraud.
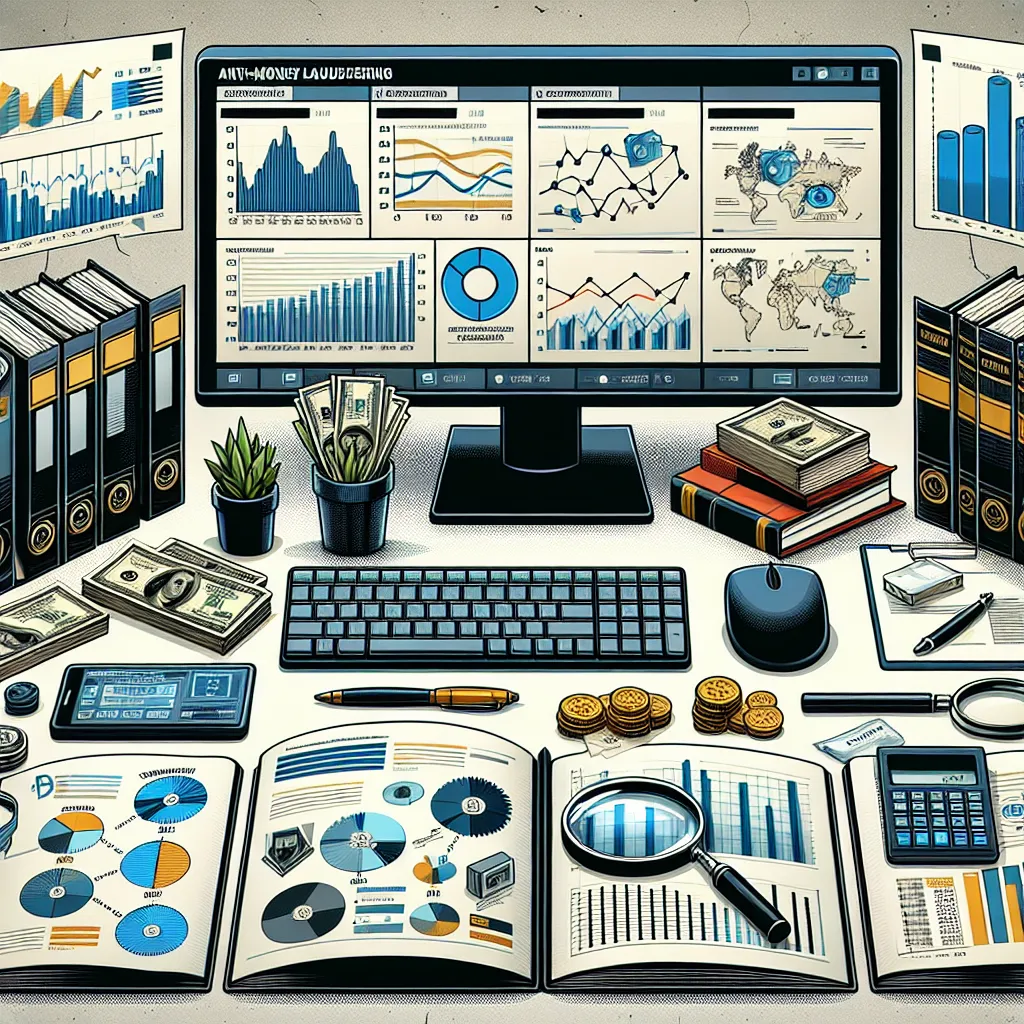
AML Transaction Monitoring Software Explained: Key Features & Best Practices
AML transaction monitoring software has become a mission-critical tool for modern financial institutions.
As financial crime grows more complex and regulators raise the bar on compliance, static or outdated systems are no longer enough. Today’s banks and fintechs need intelligent, adaptive software that not only flags suspicious activity but also learns, evolves, and reduces false positives over time. In this blog, we explore how next-generation AML transaction monitoring software works, the key features to look for, and how to future-proof your compliance operations in an increasingly dynamic threat landscape.
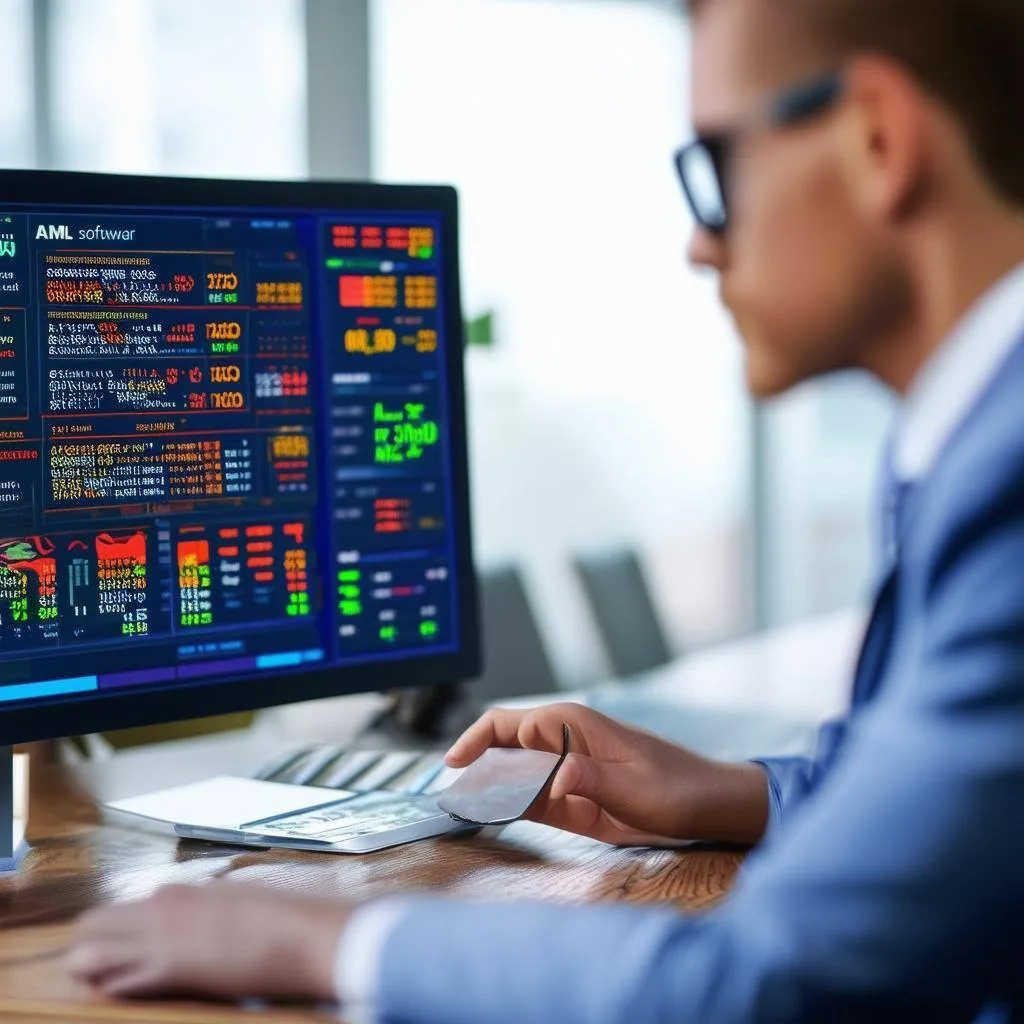
Understanding AML Transaction Monitoring Software
AML Transaction Monitoring Software is a critical tool for financial institutions, enabling them to detect and prevent illicit financial activities such as money laundering, fraud, and terrorism financing. By continuously analyzing transactional data, these systems identify unusual patterns, anomalies, and red flags that could indicate financial crime.
How Does AML Transaction Monitoring Software Work?
🔹 Real-Time Data Analysis: Advanced algorithms scan transaction data from multiple sources, instantly flagging suspicious activities. This proactive monitoring ensures risks are identified before they escalate.
🔹 Regulatory Compliance: Compliance with global AML regulations is non-negotiable. AML Transaction Monitoring Software helps financial institutions stay compliant, mitigating the risk of hefty fines and reputational damage.
🔹 AI & Machine Learning Enhancements: Traditional rule-based monitoring often leads to high false positives. AI-driven systems adapt and evolve, reducing false alarms and focusing on genuine threats with precision.
🔹 Instant Alerts & Response: The ability to detect and respond in real-time is a game-changer. Timely alerts allow compliance teams to act swiftly, preventing financial criminals from exploiting gaps in the system.
As financial crime tactics grow more sophisticated, the role of AML Transaction Monitoring Software becomes even more vital. Financial institutions that leverage AI-powered, real-time monitoring systems can stay ahead of threats while ensuring seamless compliance.
{{cta-first}}
The Importance of AML Transaction Monitoring in Financial Crime Prevention
For financial institutions, AML Transaction Monitoring Software is more than just a compliance requirement—it’s a strategic defence against financial crime. Regulatory non-compliance can lead to crippling fines and reputational damage, making robust monitoring systems essential.
Why AML Transaction Monitoring Software is Critical
🔹 Regulatory Compliance & Risk Mitigation – Global AML regulations demand continuous monitoring of transactions. A well-implemented AML Transaction Monitoring Software ensures financial institutions meet compliance requirements while minimizing legal and reputational risks.
🔹 Proven Impact on Financial Crime Prevention – Case studies show that banks leveraging AI-powered transaction monitoring solutions report a significant drop in suspicious activity. The right software enhances detection, reduces false positives, and prevents illicit transactions before they cause harm.
🔹 Seamless Integration with Other Crime Prevention Tools – Transaction monitoring doesn’t operate in a vacuum. When integrated with AI-driven fraud detection, name screening, and behavioural analytics, it creates a multi-layered defence system against evolving threats.
🔹 Big Data & AI for Smarter Detection – The ability to analyze massive data sets in real-time allows institutions to uncover hidden patterns linked to money laundering and fraud. AI and machine learning-driven AML Transaction Monitoring Software fine-tune risk detection, enabling institutions to detect sophisticated threats with greater accuracy.
With financial criminals constantly evolving their tactics, institutions that invest in advanced AML Transaction Monitoring Software stay ahead of threats, ensuring both compliance and security in an increasingly complex financial landscape.
Challenges and Solutions in Transaction Monitoring
Despite its importance, AML Transaction Monitoring Software comes with challenges that financial institutions must address to ensure accurate threat detection and compliance efficiency. From high false positives to evolving financial crime tactics, tackling these issues requires a mix of technology, fine-tuning, and expert oversight.
Key Challenges & How to Overcome Them
🔹 High Volume & Complexity of Transactions
Financial institutions process millions of transactions daily, making it difficult to distinguish between legitimate activity and illicit patterns. Solution: AI-driven AML Transaction Monitoring Software can analyze massive datasets in real-time, filtering out noise while identifying genuine threats.
🔹 False Positives Overload
Excessive false positives waste compliance resources and slow down investigations. Solution: Advanced AI/ML models continuously refine detection parameters, reducing false alerts and ensuring investigators focus on high-risk activities.
🔹 Calibrating Risk Thresholds & Parameters
Setting monitoring baselines is complex—too strict, and you trigger unnecessary alerts; too lenient, and risks go undetected. Solution: Risk-based approaches powered by behavioural analytics allow institutions to dynamically adjust thresholds based on customer profiles and transactional history.
🔹 Human Expertise & Alert Interpretation
Even the most advanced systems require human oversight. Solution: Skilled analysts are essential to differentiate between actual threats and benign anomalies. Continuous training and AI-assisted decision-making tools can enhance efficiency.
🔹 Evolving Money Laundering Tactics
Financial criminals constantly adapt to bypass detection. Solution: AML Transaction Monitoring Software must evolve too. Regular updates, AI-driven anomaly detection, and leveraging shared intelligence from compliance networks keep systems one step ahead.
The Future of AML Transaction Monitoring
As financial criminals become more sophisticated, the need for intelligent, adaptive AML Transaction Monitoring Software has never been greater. Institutions that leverage AI, machine learning, and collaborative intelligence will be best positioned to mitigate risk, improve detection accuracy, and enhance compliance effectiveness.
Key Features of AML Transaction Monitoring Systems
To effectively combat financial crime, AML Transaction Monitoring Software must be equipped with advanced capabilities that enhance detection accuracy, streamline compliance, and reduce operational inefficiencies.
Essential Features of AML Transaction Monitoring Software
🔹 Real-Time Transaction Monitoring
Instant detection of suspicious transactions is critical in preventing financial crime. AI-powered AML Transaction Monitoring Software analyzes transactions as they happen, allowing compliance teams to respond swiftly before illicit activity escalates.
🔹 Advanced Data Analytics & Pattern Recognition
With financial criminals using increasingly sophisticated laundering techniques, modern AML software leverages big data, machine learning, and behavioural analytics to detect hidden patterns and anomalies that rule-based systems might miss.
🔹 Risk-Based Customer Due Diligence (CDD & KYC Integration)
A strong AML framework starts with understanding customer behaviour. AML Transaction Monitoring Software integrates with Know Your Customer (KYC) and risk assessment systems to flag high-risk entities and enhance due diligence.
🔹 Adaptive Risk Scoring & Threshold Calibration
Static rules lead to excessive false positives or missed risks. AI-driven calibration dynamically adjusts risk thresholds based on transaction behaviour, ensuring accurate threat detection without overwhelming compliance teams.
🔹 Automated Alerts & Case Management
Efficiency is key. The best AML software provides automated alerts, case prioritization, and workflow automation, helping compliance teams focus on high-risk transactions and streamline investigations.
Why These Features Matter
In today’s fast-moving financial crime landscape, financial institutions need agile, intelligent, and scalable AML Transaction Monitoring Software to stay ahead. Leveraging these advanced features ensures stronger compliance, better fraud detection, and reduced operational burden.
Best Practices for Implementing AML Transaction Monitoring Software
Implementing AML Transaction Monitoring Software effectively requires a strategic approach that aligns with an institution’s risk profile, compliance obligations, and operational needs. Here’s how to maximize its impact:
Key Best Practices for AML Transaction Monitoring Implementation
🔹 Strengthen Customer Due Diligence (CDD)
A risk-based approach is essential for effective transaction monitoring. By analyzing customer behaviour, transaction history, and risk profiles, institutions can set precise monitoring thresholds, making it easier to detect anomalies without excessive false alerts.
🔹 Customize Software for Institutional Needs
A one-size-fits-all approach doesn’t work in AML compliance. Every financial institution has unique risks—from cross-border transactions to high-risk customer segments. AML Transaction Monitoring Software should be customizable to align with the organization’s specific risk landscape.
🔹 Train Compliance Teams Regularly
Even the most sophisticated AML software requires skilled analysts to interpret alerts and refine detection models. Continuous training ensures compliance teams stay updated on new system features, emerging financial crime tactics, and regulatory changes.
🔹 Regular System Reviews & Optimization
Financial criminals constantly evolve their tactics—so should your AML Transaction Monitoring Software. Periodic reviews, data quality assessments, and AI model recalibration help enhance detection accuracy and adapt to new threats.
Why These Best Practices Matter
By integrating these best practices, financial institutions can maximize the efficiency of their AML Transaction Monitoring Software, ensuring stronger compliance, reduced false positives, and faster response to suspicious activities.
The Future of AML Transaction Monitoring Software
As financial crime tactics grow more sophisticated, the future of AML Transaction Monitoring Software lies in predictive intelligence, regulatory adaptability, and next-gen technology. Institutions that embrace these innovations will be better equipped to prevent financial crime before it happens.
Emerging Trends in AML Transaction Monitoring Software
🔹 Predictive Analytics for Proactive Crime Prevention
The future of AML monitoring is anticipatory, not just reactive. AI-driven predictive analytics can forecast potential money laundering risks before they materialize, allowing institutions to intervene earlier and strengthen fraud prevention strategies.
🔹 Data Privacy & Protection in a Digital-First Era
With increasing global regulations on data security (e.g., GDPR, PDPA, CCPA), financial institutions must ensure AML Transaction Monitoring Software aligns with strict data protection standards. Advanced encryption, secure storage, and AI-driven anomaly detection will play a key role in securing transaction data.
🔹 Cross-Border Compliance & Regulatory Adaptability
Transaction monitoring for cross-border payments is becoming more complex due to varied AML regulations across jurisdictions. Next-gen software must integrate multi-regional compliance frameworks and AI-driven risk scoring to adapt seamlessly to different regulatory landscapes.
🔹 Blockchain & Digital Asset Monitoring
Blockchain’s transparency and immutability offer promising solutions for AML. Future AML Transaction Monitoring Software will leverage blockchain-powered monitoring to track illicit transactions across decentralized networks, improving traceability in cryptocurrency and digital asset compliance.
The Road Ahead
As financial criminals evolve, AML Transaction Monitoring Software must become faster, smarter, and more adaptive. The integration of AI, predictive analytics, blockchain, and global compliance intelligence will redefine the future of financial crime prevention.
{{cta-whitepaper}}
Conclusion: Future-Proof Your AML Compliance with FinCense
Financial crime is evolving, and compliance demands efficiency, accuracy, and scalability. Tookitaki’s FinCense delivers next-gen AML Transaction Monitoring Software, ensuring 100% risk coverage, 90% detection accuracy, and 50% lower compliance costs.
Why FinCense?
✔️ Real-time AML Transaction Monitoring – Detect and mitigate risks instantly.
✔️ AI-Powered Smart Screening & Risk Scoring – Reduce false positives by up to 90%.
✔️ Automated Case Management – Investigate customers, not just alerts, with 40% faster handling.
✔️ Seamless KYC & Onboarding Integration – Instant, risk-based customer profiling.
With FinCense, compliance teams stay ahead of threats, ensuring faster, smarter, and cost-effective AML compliance.
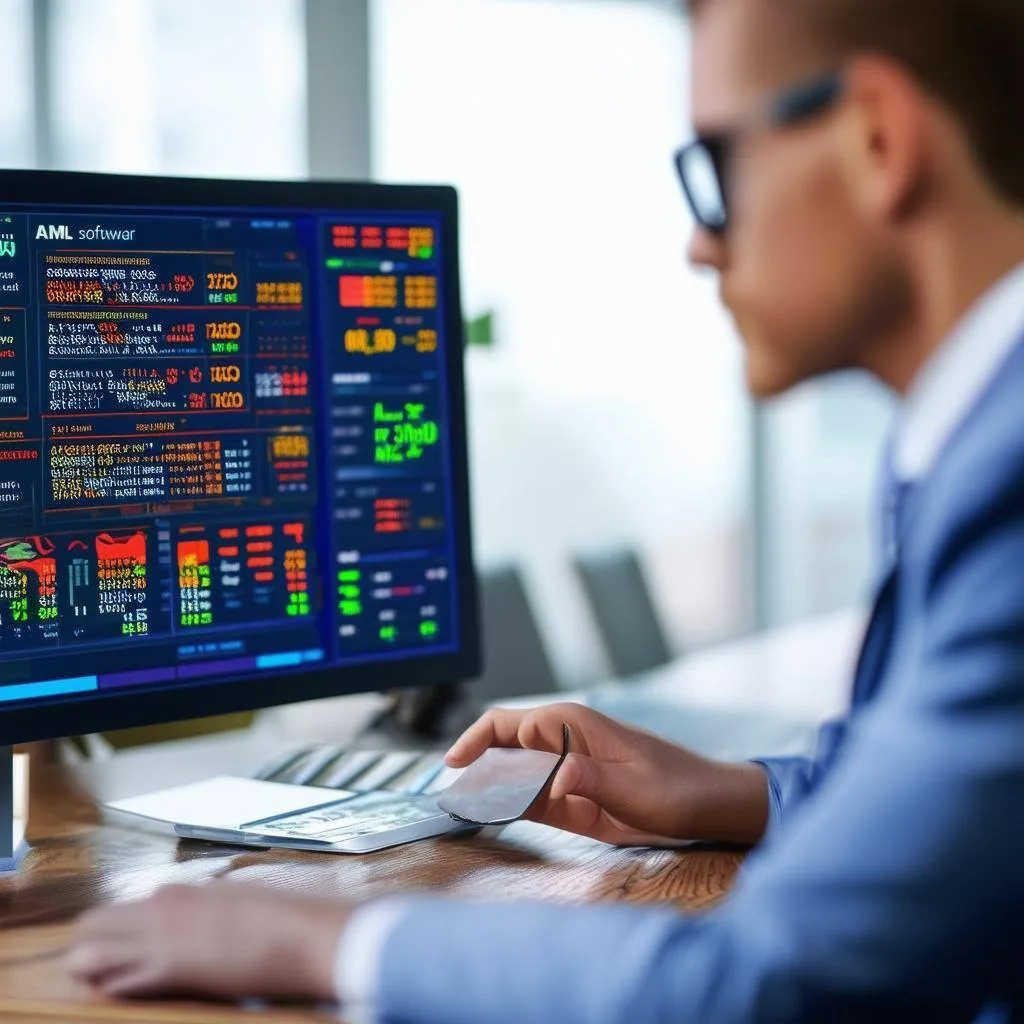
AML Fraud Detection: The Hidden Threats Banks Miss in 2025
Financial institutions worldwide face a massive challenge as criminals launder an estimated $2 trillion annually through banks. Banks pour resources into compliance programs but still miss key threats. This failure has resulted in $342 billion worth of AML fines since 2019.
The digital world of financial crime changes rapidly. Regulators have already issued 80 AML fines worth $263 million in the first half of 2024. These numbers show a 31% jump from 2023's figures. Criminals actively exploit the gaps created by banks' separate approaches to AML and fraud detection.
Banks need to understand the hidden threats they might miss in 2025. Traditional systems often fail to catch sophisticated schemes. A more integrated approach could help financial institutions protect themselves better against new risks.

The Evolution of Money Laundering Techniques in 2025
Criminal organizations keep finding new ways to commit financial crimes. Their money laundering techniques have become more sophisticated in 2025. These criminals now use complex technology-based strategies because law enforcement targets conventional methods.
Traditional vs. modern laundering methods
Money launderers used to rely on cash-heavy businesses, physical assets, and offshore accounts. Today's criminals prefer digital methods that give them better anonymity and speed. The International Monetary Fund reports that money laundering makes up about 5% of the global GDP. These numbers show how massive this criminal enterprise has become.
Modern criminals now infiltrate legitimate businesses and use complex corporate structures across borders. German authorities reported their highest financial crime damage from organized groups in 10 years during 2023. This surge proves how effective these new methods have become.
{{cta-first}}
The rise of synthetic identity fraud
Synthetic identity fraud combines real and fake information to create "Frankenstein IDs" that look genuine. This crime has become the fastest-growing financial fraud in the United States. Banks lose an estimated PHP 353.63 billion to this scheme. Each fraudulent account costs about PHP 884,063.70 on average.
These fake identities target the most vulnerable people. Criminals use children's Social Security numbers 51 times more often than others. They also target elderly and homeless people who rarely check their credit reports.
Crypto-mixing and cross-chain transactions
Cross-chain crime leads the way in cryptocurrency laundering. This technique, also called "chain-hopping," swaps cryptocurrencies between different tokens or blockchains quickly to hide their criminal sources.
Criminals have laundered PHP 412.56 billion worth of illegal crypto through cross-chain services. They prefer privacy-focused bridges like Thorchain and Incognito that use zero-knowledge proofs to hide transaction details. RenBridge alone has helped launder at least PHP 31.83 billion in criminal proceeds.
AI-powered laundering schemes
AI has changed how criminals launder money. They now use AI algorithms to create realistic fake identities, automate complex transactions, and generate convincing business documents to make illegal money look legal.
AI helps create synthetic identities for financial crimes and bypass traditional verification methods. Criminals value this technology because it automates "structured" transactions. They split large amounts into smaller transfers across multiple accounts to avoid detection systems.
Why Traditional AML Systems Fail to Detect New Threats
Banks invest heavily in compliance but still struggle to catch sophisticated money laundering schemes. Their existing systems can't keep up with new criminal tactics. This creates dangerous blind spots that lead to billions in fines.
Rule-based limitations in complex scenarios
AML systems today depend too much on fixed rules and thresholds that criminals know how to bypass. These rigid systems flood analysts with false alarms, which makes real threats harder to spot. A Chief AML Officer at a financial institution learned they could turn off several detection rules without affecting the number of suspicious activity reports.
Rule-based monitoring has a basic flaw - it can't place transactions in context. The system doesn't know the difference between a pizza delivery worker getting drug money from another state and a student receiving help from family. This makes investigators tune out alerts and miss actual suspicious activity.
Data silos preventing holistic detection
Teams that don't share information make it harder to catch financial crimes. Research shows 55% of companies work in silos, and 54% of financial leaders say this blocks progress. The cost is staggering - Fortune 500 companies lose PHP 1856.53 billion each year by not sharing knowledge between teams.
The Danske Bank scandal shows what can go wrong. The bank couldn't combine its Estonian branch's systems with main operations, which left a gap where suspicious transactions went unnoticed for years. Important data stuck in separate systems or departments makes compliance work slow and prone to mistakes.
Outdated risk assessment models
Most banks still use basic customer risk profiles that quickly become stale. They collect information when accounts open but rarely update it. Banks expect customers to refresh their own details, which almost never happens.
Old-style risk tools built on spreadsheets and static reports can't handle large-scale data analysis. This limits their ability to spot patterns that could paint a better risk picture. Many banks only check risk once a year - a process that drags on for months. Criminals exploit this gap between their new methods and the bank's outdated models.
Hidden Threats Banks Are Missing Today
Financial institutions can't keep up with evolving money laundering tactics that exploit gaps between traditional AML and fraud detection systems. Criminals move billions undetected by using sophisticated threats that operate in detection blind spots.
Smurfing 2.0: Micro-transactions across multiple platforms
Traditional "smurfing" has grown beyond breaking large transactions into smaller ones. Criminals now spread tiny amounts across many digital channels in what experts call "micro-money laundering." They avoid suspicion by making hundreds of small transactions that look legitimate on their own.
This approach works well because:
- Digital payment platforms enable quick, high-volume, small-value transactions
- Alert systems miss these micro-transfers since they stay below reporting limits
- Spreading transactions across platforms prevents banks from seeing the full picture
Legitimate business infiltration
Criminal networks in the EU have found a new way to hide their activities - 86% now use legal business structures as cover. Cash-heavy businesses make perfect fronts for laundering money and create unfair advantages that hurt honest companies.
Criminals naturally blend legal and illegal operations through high-level infiltration or direct ownership. Some companies exist purely as fronts for criminal activities, while bad actors buy others to achieve their long-term criminal goals.
Real-time payment exploitation
Real-time payments give fraudsters the perfect chance to strike. These transactions can't be reversed once started, which leaves banks no time to step in. Fraud losses jumped 164% in just two years after real-time payment services launched in the US and UK.
Banks struggle to keep pace with these systems that process transactions around the clock. The risk grows since delayed detection means criminals have already moved the money before anyone spots the fraud patterns.
Mule account networks
Modern money laundering operations rely heavily on sophisticated mule networks. Between January 2022 and September 2023, just 25 banks removed 194,084 money mules from their systems. The National Fraud Database only received reports for 37% of these accounts.
Mule handlers recruit people to move dirty money through personal accounts. This creates complex patterns that hide the money's true path. Many banks still can't detect customers who knowingly join these schemes, especially when transactions appear normal on the surface.
AML vs Fraud Detection: Bridging the Critical Gap
Financial institutions have managed to keep separate teams to fight fraud and money laundering. This setup creates dangerous gaps in their defensive armor. Criminal operations now blur the lines between fraud and laundering activities, which makes us think about these long-standing divisions.
Understanding the fundamental differences
AML and fraud detection work differently within financial institutions. Chief Compliance Officers watch over AML as a compliance-driven operation. Meanwhile, Chief Risk Officers handle fraud detection as a risk management function. The main difference shows in their focus. AML stops criminals from making illegal money look legitimate. Fraud prevention protects customers and institutions from losing money.
Their approaches work quite differently:
- Fraud monitoring uses live detection to stop fraud before it hits customers
- AML monitoring looks at detailed data analysis to spot suspicious patterns and meet legal requirements
Where traditional approaches create blind spots
Separate teams create major weak points in the system. Money laundering usually follows fraud, but most institutions look at these risks separately. This separation leads to:
- Teams doing the same alert reviews and case investigations twice
- Risk assessment models that can't see connected activities
- Resources, systems and data management that don't work well together
Separate approaches miss a key point: fraudulent transactions often point to money laundering activity. This needs suspicious activity reports even without clear connections.
The FRAML approach: Integrated protection
FRAML (Fraud Risk Assessment and Management Lifecycle) brings together fraud management and AML principles into one framework. This integrated way shows that these financial crimes share common patterns and risk factors.
The benefits show up quickly:
- Risk assessments that look at both fraud and money laundering threats
- Teams share data analytics and investigations to spot suspicious transactions faster
- Companies can save 20-30% through better systems and processes
Case study: How integration caught what siloed systems missed
A prominent North American Tier 1 bank tried a FRAML analytics approach. They fed data from multiple sources into one accessible interface. These sources included fraud detection, KYC, documentation, sanctions, and transaction monitoring. This change helped them catch 30% more mule accounts in just one year.
A mid-tier payments startup saw similar results. They improved their work output by 20% after bringing fraud and AML detection together. Their team projects that this number could reach 40% over the next year.
{{cta-whitepaper}}
Conclusion
Criminal money laundering methods have evolved beyond what traditional detection systems can handle. Banks that keep their AML and fraud detection systems separate create weak spots that criminals actively target.
Banks need complete solutions to connect fraud prevention with AML compliance. The FRAML approach works well - early users have seen their threat detection improve by 30%. Tookitaki's AFC Ecosystem and FinCense platform deliver this integrated protection. They merge up-to-the-minute intelligence sharing with complete compliance features.
Financial institutions can now better shield themselves from new threats like synthetic identity fraud, crypto-mixing, and complex mule account networks. Both large banks and payment startups have proven the worth of unified systems. Their success stories show better detection rates and budget-friendly results through optimized operations.
The battle against financial crime demands continuous adaptation and alertness. Traditional methods are not enough as criminals keep improving their tactics. Banks must accept new ideas that combine advanced analytics, live monitoring, and community-driven intelligence to remain competitive against evolving threats in 2025 and beyond.
