Success Tale: Setting a New Benchmark for AI-based AML Compliance
Tookitaki achieved a rare and historic milestone as our Anti-Money Laundering Suite (AMLS) solution went live within the premises of United Overseas Bank (UOB), one of the top 3 banks in Singapore. We became the first in the APAC region to deploy a complete AI-powered anti-money laundering (AML) solution in production concurrently to two AML risk dimensions, namely transaction monitoring (TM) and name screening (NS). By deploying Tookitaki’s AI-enabled AMLS, UOB could effectively create workflows for prioritizing TM and NS alerts based on their risk levels to help the compliance team focus on those alerts that matter the most. Vindicating the efficacy, robustness and sustainability of the machine learning models involved, AMLS underwent multiple rounds of rigorous testing, validation and evaluation, involving third-party consultants, before going live in full scale.
Compliance Challenges That Prompted us to be Innovators
Combating money laundering has become an enormous task for financial institutions, and it comes with substantial costs and risks, including but not limited to regulatory, reputational and financial crime risks. During the first half of 2020, APAC regulators imposed almost USD 4 billion in fines for AML violations, according to a report. Ineffective risk-based frameworks, deficient monitoring systems, inadequate review of suspicious activity, and unoptimized resources allocation are some of the widely cited AML compliance problems for financial institutions.
A leading bank in Southeast Asia with a global network of more than 500 offices in 19 countries and territories in Asia Pacific, Europe and North America, UOB wanted to have a holistic view of money laundering risks and the threat-scape across various banking segments such as corporate, retail and private. Existing static and granular rules-based approaches, which are oblivious of the holistic trend with a narrow and uni-dimensional focus, were not capable of doing the same. For UOB, which is handling about 30 million transactions and more than 5,700 TM alerts per month, existing rules-based systems produced a significant volume of false positives. The situation was not different in the case of the NS process, where the bank screened about 60,000 account names on a monthly basis. These false leads are a drain on productivity as they take significant time and resources to be disposed of. In the AML compliance space, banks are wasting more USD 3.5 billion per year chasing false leads because of outdated AML systems that rely on stale rules and scenarios and generate millions of false positives, according to research.
Undoubtedly, using limited resources to close off non-material and unimportant alerts is manual and onerous, resulting in huge backlogs for both processes and missed/delayed Suspicious Activity Report (SAR) filings. Furthermore, the ballooning costs of AML compliance coupled with the high volume of backlog alerts swamp compliance teams and potentially distract them from ‘true’ high-risk events and customer circumstances. Alert investigation was a time-consuming and labour-intensive affair as the compliance team spent significant time in gathering data and analysing it to differentiate illegitimate activities from legitimate ones. Disparate data sources and highly complex business processes added to the difficulty of the investigation team in analysing the links between parties and transactions.
These issues prompted the bank to leverage innovation and next-generation technology to enhance existing AML compliance processes, surveillance systems, and alert handling practices. In specific, UOB wanted a next-gen solution that can do the following:
- Identification of non-material false positives for both TM and NS using data from disparate sources.
- Accurate grouping of high-risk alerts for increased focus by compliance personnel.
- Advanced analytics combining data from existing financial crime systems and numerous disparate data sources.
- Faster investigation and resolution of all alerts by connecting the dots within the data, and constructing a more holistic global view of accounts, counterparties and transactions, effectively reducing the high volume of alert backlogs.
AMLS: An Innovation Proven for Robustness, Agility and Sustainability
As part of its ‘AML/CFT Technology Roadmap’ to harness next-generation AI and machine learning-driven technologies to combat money laundering, UOB teamed up with Tookitaki. The bank’s aspiration was to shift beyond rules-based systems to achieve higher performance with machine learning models and other disciplines of AI. Tookitaki’s ability to seamlessly connect with existing AML systems at UOB for data ingestion hastened the bank’s decision to onboard us.
As such, Tookitaki developed AMLS, an end-to-end AML compliance solution that combines supervised and unsupervised machine learning techniques to detect suspicious activities and identify high-risk clients quicker and more accurately. We use a combination of machine learning algorithms to build highly accurate and stable models and techniques such as dynamic clustering which does behavioural segmentation based on composite features. AMLS TM module can prioritise known alerts based on their risk scores and detect new, unknown suspicious patterns. The NS module has three core components – enhanced name matching through a wider range of complex name permutations, reduction of undetermined hits through inference features and accurate alert detection through primary and secondary information. These capabilities help accurately distinguish between false hits and true hits. The major innovative features of the solution are:
- Smart Alert Triage: The solution offers a smart way to triage TM and NS alerts by segregating them into three risk buckets – L1, L2 and L3 – where L3 is the highest-risk bucket. The highly accurate alert classification helps UOB’s compliance team to allocate time and experience judiciously and effectively address alert backlogs. Compliance analysts can now focus on those high-risk cases (L3 and L2) that require more time to investigate and close. Meanwhile, they can close low-risk alerts (L1) with minimal investigation. AMLS generates a probability score for all alerts, along with an explanation to guide the investigator make the right decision faster.
- Champion–Challenger Approach: A core component of our data science platform, this approach enables machine learning models to continuously learn from data shifts and data additions. It helps ensure that the model remains effective and unbiased amid incremental changes in data.
- Explainable AI (XAI) Framework: Our patent-pending XAI framework provides transparent machine learning models, and explainable and documentable predictions to ensure thorough understanding and to conduct quality investigations along with aligning users with the compliance model transparency requirements of regulators.
- Scalability: AMLS uses a combination of distributed data-parallel architecture and machine learning to ensure scalability across the bank’s multiple business lines and complex layers of existing technologies and systems.
Unique Implementation Approach Resulting in Sustained Model Performance
UOB had tested the effectiveness of AMLS in terms of alert prioritization in a six-month pilot started in early 2018. After receiving successful results, which Deloitte validated, the bank tested the solution again with a unique data set and performed another round of model validation. The subsequent machine-learning models outperformed the results we achieved during the pilot. The successful results gave UOB the confidence to move the machine learning models to production and build a tailored solution. Based on the bank’s feedback, Tookitaki introduced various enhancements and additional features into its solution.
While deploying AMLS on UOB premises, we took a unique approach of augmenting existing systems with AI-based smart alert management where our solution would sit on top of existing TM and NS solutions and accurately group alerts for faster closure. In the model training phase, our solution’s powerful integration layer extracted data from existing product systems and primary TM and NS systems, transformed them and then loaded them to our platform. This used to be a process that requires considerable effort and time, however, Tookitaki solution’s pre-packed connectors made it easier for us to adapt to the bank’s various enterprise architectures and up-stream systems.
For TM execution, we integrated historical data for three years (customer, accounts, transactions, primary system alerts, etc.) in the learning phase. In NS, which is used to identify individuals and entities that are involved in AML activities, our advanced name matching algorithms compared individual names and business names with the bank’s internal and external watch lists. Our solution could effectively handle multiple attributes such as typos, transliteration limitations, cultural differences for accurate hits detection.
After validating the accuracy and stability of the training models, we moved to the execution mode where we integrated additional data from source systems. The final models used in TM and NS processes helped execute alert prioritization accurately and investigate alerts in a faster manner. AMLS consolidated all source data to provide a holistic view of customers, accounts and transactions and brought in enhanced network analysis and intelligent cluster analysis to aid investigative functions across various business units within the bank.
The business interface of AMLS provides easy-to-use and highly customizable dashboards for both TM and NS processes, enabling efficient work allocation, exploratory analysis, link analysis, prediction interpretation and management reporting.
The following are the quantitative business benefits we received from the project.
- Name Screening: 70% reduction in false positives for individual names and 60% reduction in false positives for corporate names.
- Transaction Monitoring: 50% reduction in false positives with less than 1% misclassification, 5% increase in true positives (file-able SARs) and an overall true positive prediction rate of 96% in the high-priority category.
Other benefits we achieved are:
- Increased effectiveness in identifying suspicious activities
- A sharper focus on data anomalies rather than depending on threshold triggering
- Easier customisation of data features to target specific risks accurately
- Ability to enable longer look-back periods to detect complex scenarios
Protecting against model biases, our platform’s Champion-Challenger module automatically and continuously incorporates data shifts and data additions and informs users of the availability of any ‘Challenger’ model. Users may validate the vitals of the newly created ‘Challenger’ and replace it with the existing ‘Champion’ effortlessly. This unique feature helps financial institutions avoid time-consuming and costly model upgrades, ensuring faster ROI realization and sustained and effective performance of AML compliance programs.
The deployment of AMLS at UOB with stellar results marks the end of the AI experimentation phase in AML compliance. It is another example of how Tookitaki, as a fast-growing AI startup, sets new standards for the regulatory compliance industry’s fight against money laundering. Our success is noteworthy given that many enterprise AI projects are dying within laboratories. AMLS went through multiple rounds of testing and validation and our machine learning models have been proven to provide stable results and remain agile to the cause in dynamic situations. At the same time, it could effectively explain the decision-making process of machine learning models in a comprehensive yet simple manner through our patent-pending Explainable AI framework. Through this project, we also validated that our AI processes are effective, efficient and set to be applied in a responsible and ethical manner.
A complete revamp of existing AML compliance processes is imperative for financial institutions, given that money laundering strategies are becoming more and more sophisticated. It is time to embrace modern-era intelligent technology to enhance efficiency and effectiveness in AML compliance programs, establish next-gen financial crime surveillance and ensure robust risk management practices.
For more details about our partnerships with UOB and many other big banks across the globe, please contact us.
Experience the most intelligent AML and fraud prevention platform
Experience the most intelligent AML and fraud prevention platform
Experience the most intelligent AML and fraud prevention platform
Top AML Scenarios in ASEAN

The Role of AML Software in Compliance

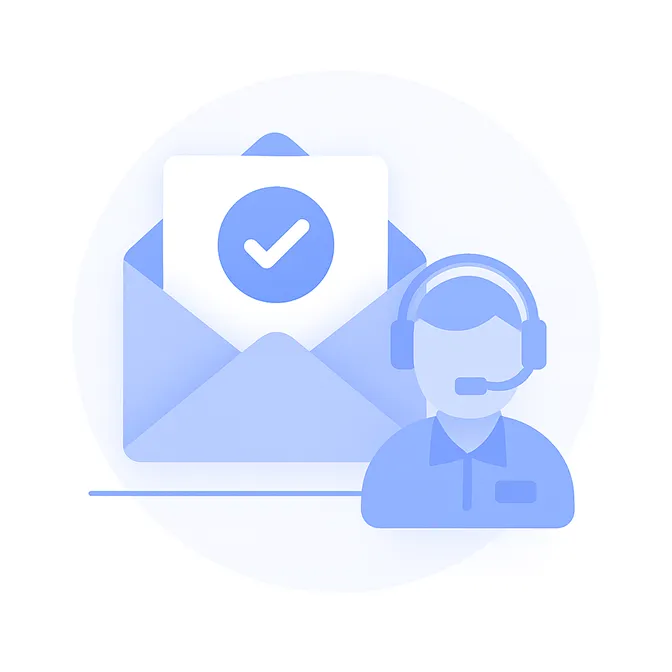