Pitfall of Black Box AI at Banks: Explaining Your Models to Regulators
The use cases of artificial intelligence (AI) and machine learning in front-office, middle-office and back-office activities at banks are growing slowly but steadily. The major areas of AI play include customer service (virtual assistants, chatbots, etc.), fraud detection, risk management, predictive analytics, and automation. Like in any other industries, AI, if implemented the right product in the right manner, can increase the efficiency of banking operations as well as reduce their cost (up to more than USD 1 trillion by 2030, according to experts). Of course, there are problems related to distinct data sets and data privacy that curtail the implementation of these technologies. However, AI would turn into a new normal at banks as existing workflows are set to become unsustainable due to the ever-increasing scale of operations. These days, most banks are operating round the clock due to the emergence of online banking and mobile banking. Along with that, the financial inclusion initiatives across the globe would see a gigantic rise in the volume of banking operations. Therefore, banks would require rapid processing abilities to stay relevant and ensure the satisfaction of various stakeholders including customers and regulators.
Though the field is somewhat set for AI at banks with the advent of mobile technology, data availability and abundance of open-source APIs, there are certain systemic problems that banks are concerned about. Banks are worried if their regulators would accept the use of technologies, which are relatively new and different from the existing ones to a great extent. There are also risks related to possible biases in machine learning algorithms due to data quality and data accuracy. Black box AI algorithms are another concern that can hinder the adoption of AI in banking. Here, we are trying to explain the concept of black box AI, its problems and how banks can overcome the challenge.
What is black box AI?
Black box AI is a problem in machine learning where even the designers of an algorithm cannot explain why and how it arrived at a specific decision. The fundamental problem here is: if we cannot figure out how AI has come up with its decisions, how can we trust AI? This trust issue led to the failure of IBM Watson (especially (Watson for Oncology), one of the best-known AI innovations in recent times. The main problem with a black box model is its inability to identify possible biases in the machine learning algorithms. Biases can come through prejudices of designers and faulty training data, and these biases lead to unfair and wrong decisions. Bias can also happen when model developers do not implement the proper business context to come up with legitimate outputs.
The same problem is relevant in the banking industry as well. If regulators pose a question: how AI has reached at a conclusion with regard to a banking problem, banks should be able to explain the same. For example, if an AI solution dealing with anti-money laundering compliance comes up with an anomalous behaviour or suspicious activity in a transaction, the bank using the solution should be able to explain the reason why the solution has arrived at that decision. Such an audit is not possible with a black box AI model. The same concern was expressed by Federal Reserve Gov. Lael Brainard in a November 2018 speech. “AI can introduce additional complexity because many AI tools and models develop analysis, arrive at conclusions, or recommend decisions that may be hard to explain. For instance, some AI approaches are able to identify patterns that were previously unidentified and are intuitively quite hard to grasp. Depending on what algorithms are used, it is possible that no one, including the algorithm's creators, can easily explain why the model generated the results that it did,” she said.
Not just AI, Banks need explainable AI
Explainable AI or interpretable AI or transparent AI deals with techniques in artificial intelligence which can make machine learning algorithms trustworthy and easily understandable by humans. Explainability has emerged as a critical requirement for AI in many cases and has become a new research area in AI. As mentioned by the Defense Advanced Research Projects Agency under the US Department of Defense: “New machine-learning systems will have the ability to explain their rationale, characterize their strengths and weaknesses, and convey an understanding of how they will behave in the future.”
In the banking industry, which is subject to stricter regulatory oversight across the globe, an incorrect decision can cost billions of dollars for an institution. If a bank wants to employ AI, it is imperative for it to subject the particular solution to rigorous, dynamic model risk management and validation. The bank must ensure that the proposed AI solution has the required transparency depending on the use case. As an AI solutions provider, Tookitaki has always considered explainability as a must-have feature in its offerings. Its unique technology demystifies modern machine learning and gives clients the knowledge and tools to outperform the competition. Tookitaki solutions feature a ‘Glass box’ audit module that brings algorithmic transparency by providing thorough explanations for predictions.
There is no doubt that AI can bring in revolutionary changes in the banking sector. For that to happen, it is mandatory that banks should take the necessary oversight to prevent their AI models from being a black box. As of now, the AI use cases are mostly in low-risk banking environments, where human beings still take the final decision with machines just providing valuable assistance in decision making. In future, banks will be under pressure to remove some of the human oversight for cost savings amid increasing scale of operations. At that point, banks cannot run with risky black box models that can lead to inefficiencies and risks. They need to ensure that their AI solutions are trustworthy and have the required transparency to satisfy internal and external audits. In short, the bright future of AI in banking could be assured only through explainable AI.
Experience the most intelligent AML and fraud prevention platform
Experience the most intelligent AML and fraud prevention platform
Experience the most intelligent AML and fraud prevention platform
Top AML Scenarios in ASEAN

The Role of AML Software in Compliance

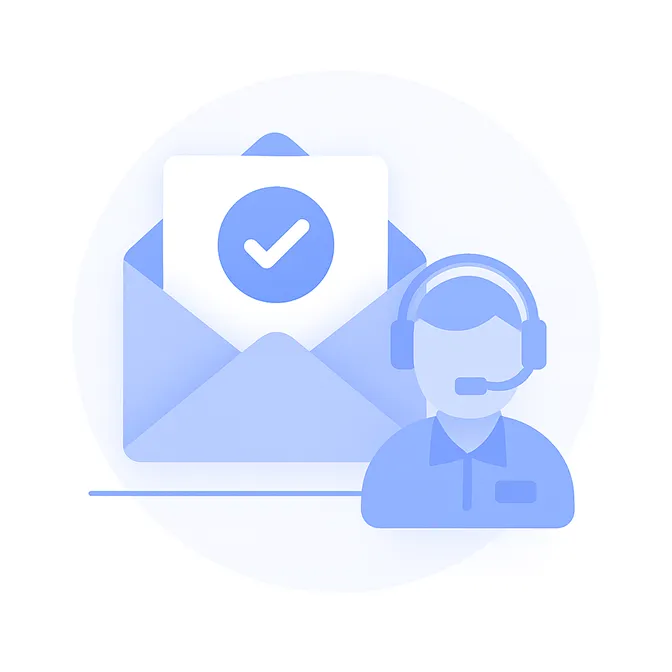
We’ve received your details and our team will be in touch shortly.
Ready to Streamline Your Anti-Financial Crime Compliance?
Our Thought Leadership Guides
Thailand’s AML/CFT Wake-Up Call: What Banks and Fintechs Must Prepare for in 2025
Thailand’s financial system is entering a defining era for anti-money laundering and counter-terrorism financing.
As the country deepens its regional trade and digital finance ambitions, it also faces mounting pressure to confront evolving financial crime threats, ranging from cross-border laundering to high-velocity scams and informal value transfers. With the FATF eyeing gaps in oversight and regulators tightening expectations, AML/CFT compliance is no longer just a back-office responsibility. It's a front-line defence for trust and competitiveness.
In this blog, we break down the current AML/CFT regulatory framework in Thailand, highlight key risks and real-world threats, explore upcoming reform pressures, and share how banks and fintechs can strengthen their compliance strategy through both innovation and collaboration.
The Regulatory Landscape in Thailand
Thailand’s AML/CFT framework is governed by the Anti-Money Laundering Office (AMLO), established in 1999. AMLO functions as both the financial intelligence unit (FIU) and the key enforcement agency overseeing compliance and investigations related to illicit finance.
The two core laws forming the backbone of the regulatory regime are:
- The Anti-Money Laundering Act (AMLA), B.E. 2542 (1999)
- The Counter-Terrorism and Proliferation of Weapons of Mass Destruction Financing Act, B.E. 2559 (2016)

Entities subject to AML/CTF obligations include:
- Commercial banks and financial institutions
- Money service businesses (MSBs), e-wallets, and fintech platforms
- Securities companies and insurance providers
- Real estate developers and dealers in precious stones/metals
- Legal professionals and notaries (in limited contexts)
Reporting entities must:
- Conduct customer due diligence (CDD) and enhanced due diligence (EDD)
- File suspicious transaction reports (STRs) and cash transaction reports (CTRs) with AMLO
- Retain records for a minimum of 5 years
- Establish internal AML programs, risk assessments, and staff training
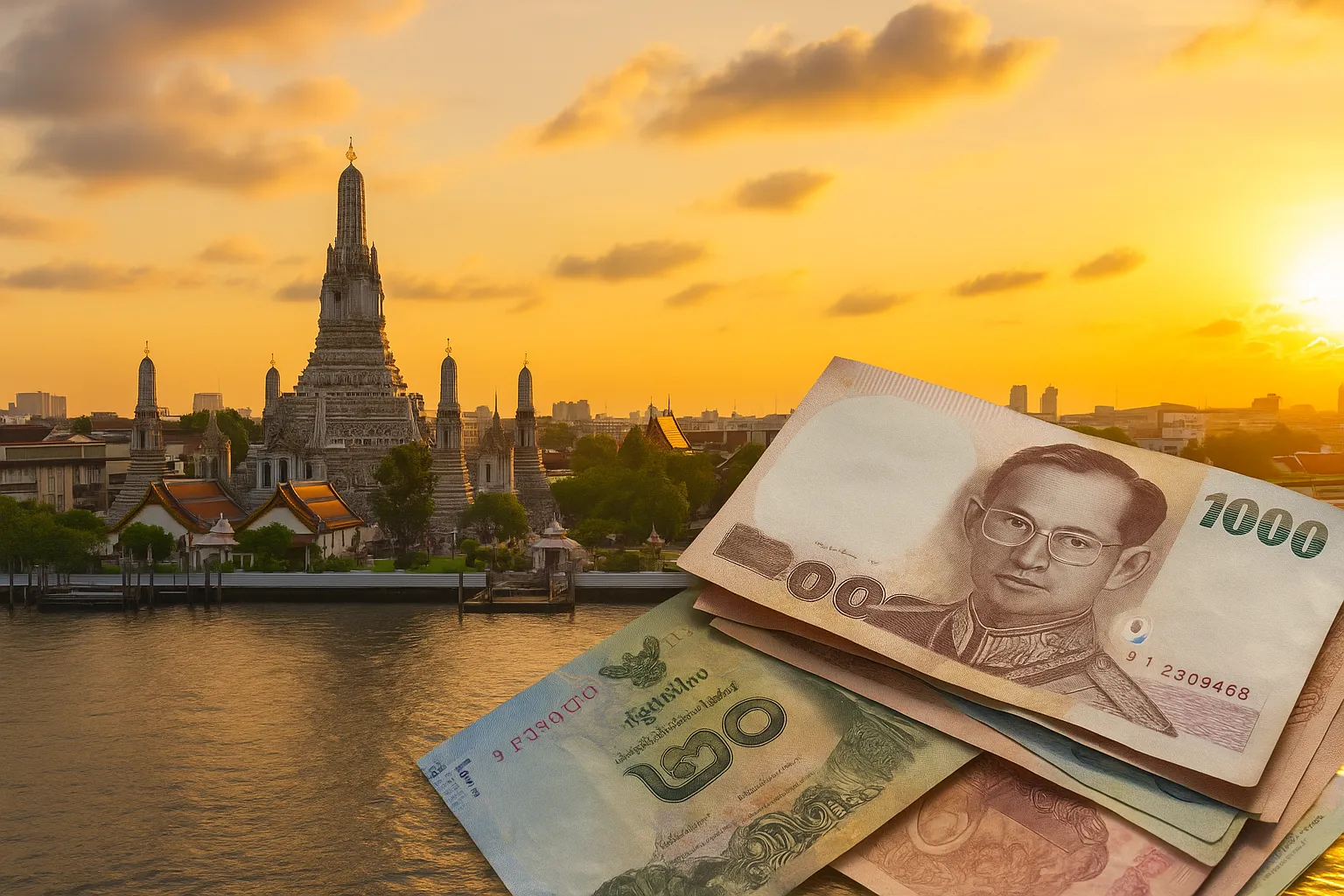
FATF and Grey List Pressures
Thailand has had a complicated relationship with the Financial Action Task Force (FATF). After being grey-listed in 2011 due to strategic deficiencies in its AML regime, it made significant reforms and was removed in 2015.
However, FATF’s most recent mutual evaluation pointed to new challenges:
- Limited oversight of certain non-financial sectors
- Gaps in beneficial ownership transparency
- Uneven application of risk-based approaches
- Under-reporting of suspicious transactions by fintech and digital players
Why it matters: FATF grey-listing carries serious consequences. It can deter foreign investment, slow correspondent banking relationships, and increase the cost of doing business internationally. For Thai banks and fintechs, staying aligned with FATF expectations is not just about compliance—it’s about global competitiveness.
Real-World Threats: What’s Fueling Financial Crime in Thailand
Thailand’s economy, geographic location, and strong informal networks make it vulnerable to a wide range of predicate offences. Some of the most prominent financial crime threats include:
🔹 Drug Trafficking and Organised Crime
Transnational criminal groups exploit Thailand’s location in the Mekong subregion to launder drug proceeds through shell companies, property purchases, and trade channels.
🔹 Public Sector Corruption and Tax Crimes
Illicit enrichment and VAT fraud are common predicate offences, with funds often laundered via nominee accounts and luxury assets.
🔹 Cross-Border Laundering
Money mules, informal remittance systems, and trade-based money laundering (TBML) remain significant threats. Syndicates frequently layer funds through multiple jurisdictions.
🔹 Investment and Romance Scams
Thailand is increasingly being used as both a staging ground and a destination for proceeds from international fraud, including pig butchering scams and tech support frauds targeting foreign victims.
AMLO has flagged the rising use of e-wallets, digital platforms, and cash-intensive businesses as high-risk vehicles for laundering.
Challenges for Banks and Fintechs
Despite progress, many institutions face real hurdles when it comes to AML/CFT execution.
- Legacy Systems and Manual Workflows
Traditional rule-based systems often generate high false positives and miss nuanced patterns, especially in real-time transactions. - Fragmented Intelligence
Limited cross-institutional data sharing weakens the detection of syndicated risks, such as mule networks operating across multiple banks. - High Compliance Costs
Smaller fintechs and non-bank financial institutions struggle to meet regulatory requirements without draining operational resources. - Speed vs Safety in Payments
Instant payment rails (e.g., PromptPay) have made fund movement frictionless, but also difficult to trace once fraud or laundering occurs.
Thailand’s Push Toward RegTech and AI
Recognising these challenges, regulators and industry players are increasingly turning to RegTech to strengthen compliance without stifling innovation.
Notable trends:
- AI-driven transaction monitoring is gaining traction for detecting suspicious behaviour across vast datasets in real time.
- Automated screening tools are being used to process watchlists, sanctions, and politically exposed person (PEP) data more efficiently.
- Digital KYC and eKYB (Know Your Business) solutions are helping fintechs onboard customers with less friction and more accuracy.
AMLO itself has been vocal about the importance of technology in enhancing reporting accuracy and enabling real-time intelligence. Collaboration between regulators and the private sector on typology sharing and case-based learning is also gaining momentum.
How Tookitaki Supports Smarter Compliance in Thailand
Tookitaki’s FinCense platform is well-positioned to help Thai banks and fintechs overcome both regulatory and operational AML/CFT challenges.
Here’s how:
🔹 Scenario-Based Detection
FinCense leverages typologies contributed by global experts through the AFC Ecosystem. These include real-world cases such as QR-code laundering, mule account recruitment, and shell invoicing many of which mirror red flags identified by AMLO.
🔹 Smart Screening
Advanced screening tools that support multi-lingual names, alias logic, and national ID handling—critical in jurisdictions like Thailand.
🔹 AI-Powered Risk Scoring
Dynamic customer risk scoring and automated threshold tuning reduce false positives and allow institutions to focus on the most relevant alerts.
🔹 FinMate: AI Copilot for Compliance Teams
FinMate assists investigators by summarising alerts, surfacing behavioural insights, and recommending next steps, reducing the average case investigation time.
Whether you're dealing with fraud from romance scams or laundering via e-wallet networks, FinCense offers a flexible, modular approach that’s ready for Thailand’s fast-evolving risk environment.
Key Takeaways for Compliance Teams
✅ Thailand’s AML/CFT ecosystem is evolving, but financial crime threats are getting more sophisticated.
✅ FATF scrutiny and regulatory reform will intensify over the next 12–18 months.
✅ Manual systems are no longer sustainable—technology is a must-have.
✅ Cross-border risk requires cross-sector intelligence—collaboration is key.
✅ Institutions that prioritise adaptive compliance now will gain a strategic edge in the future.
%2520(1).webp)
Conclusion: Thailand’s Next Chapter in AML/CFT Compliance
Thailand has made significant progress in building its AML/CFT regime, but the fight is far from over. As financial crime networks grow more organised and tech-savvy, regulators and institutions must respond in kind—with smarter systems, stronger collaboration, and a proactive mindset.
The future of compliance in Thailand isn’t just about ticking regulatory boxes. It’s about building trust, resilience, and readiness—not just for the next audit, but for the next threat.
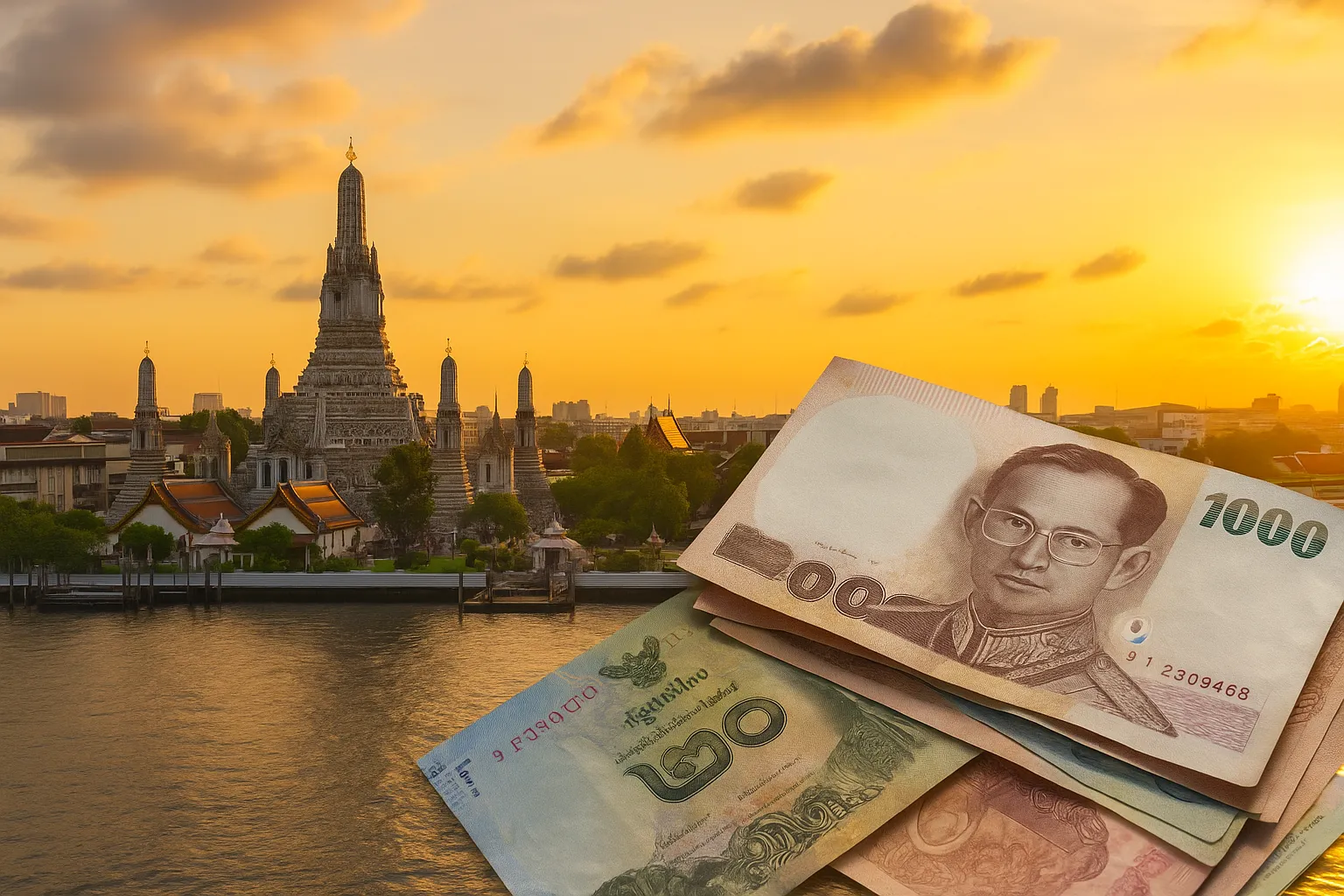
Inside AUSTRAC: Navigating Australia’s AML/CTF Regulations in a High-Risk Era
As money laundering methods grow more sophisticated, the pressure on financial institutions to detect, report, and prevent financial crime is intensifying — and AUSTRAC is at the centre of it all.
In an era where financial ecosystems are rapidly digitising, AUSTRAC’s role in overseeing Anti-Money Laundering (AML) and Counter-Terrorism Financing (CTF) compliance has become mission-critical. For banks, fintechs, and other reporting entities, staying ahead of regulatory expectations is no longer just a compliance issue — it’s a matter of reputation, trust, and long-term viability.
In this blog, we explore:
- AUSTRAC’s mandate and structure
- Key AML/CTF obligations under Australian law
- Landmark enforcement cases
- Upcoming reforms, including Tranche 2
- FATF scrutiny and global compliance pressures
- How tech-forward compliance strategies are reshaping the future

What is AUSTRAC and Why Does It Matter?
AUSTRAC — the Australian Transaction Reports and Analysis Centre — is the government body responsible for detecting and disrupting criminal abuse of Australia’s financial system.
AUSTRAC has a dual mandate:
- Regulator: Supervises compliance with AML/CTF obligations.
- Financial Intelligence Unit (FIU): Collects and analyses data to support law enforcement, national security, and international counterparts.
It works with over 17,000 reporting entities, ranging from traditional banks to digital wallets, remittance providers, gaming platforms, and more. As both a data collector and enforcer, AUSTRAC is uniquely positioned to uncover illicit financial activity at scale.
A Brief History of AML/CTF Regulation in Australia
Australia’s journey in strengthening its anti-money laundering and counter-terrorism financing framework began in earnest with the passage of the AML/CTF Act in 2006. This legislation introduced foundational obligations such as KYC procedures, transaction monitoring, and reporting requirements for a wide range of financial institutions and service providers.
Over time, the regime has evolved significantly. In 2014, AUSTRAC formalised the risk-based approach, requiring entities to tailor their AML programs based on their specific exposure to financial crime risks.
The period between 2018 and 2020 marked a turning point in enforcement, with AUSTRAC taking decisive action against some of Australia’s largest institutions — including Tabcorp, the Commonwealth Bank, and Westpac — for major compliance failures.
In the years that followed, Tranche 2 reforms were proposed to expand AML/CTF obligations to include professions such as lawyers, accountants, and real estate agents, which are known to be exploited for laundering illicit funds.
As of 2024, these reforms remain under active discussion, with the Australian government under growing pressure from international bodies such as the FATF to close regulatory gaps. The expected passage of Tranche 2 in 2025 would significantly broaden AUSTRAC’s regulatory reach and bring Australia closer in line with global AML standards.

Understanding Your AML/CTF Obligations
If your institution provides “designated services” under the AML/CTF Act, here’s what you’re required to do:
🔹 AML/CTF Program (Part A and Part B)
- Part A: Institutional risk assessments, governance, reporting, and training
- Part B: Customer identification and verification procedures (KYC)
🔹 Reporting Requirements
- Suspicious Matter Reports (SMRs)
Must be submitted when the activity raises suspicion, regardless of the amount. - Threshold Transaction Reports (TTRs)
For cash transactions of AUD 10,000 or more. - International Funds Transfer Instructions (IFTIs)
Mandatory for cross-border fund movements.
🔹 Customer Due Diligence (CDD)
- Verify customer identity at onboarding
- Apply Enhanced Due Diligence (EDD) for high-risk customers or transactions
- Conduct ongoing monitoring
🔹 Record Keeping
- Maintain transaction and identity verification records for at least 7 years.
AUSTRAC’s Enforcement Power: Learning from Past Failures
AUSTRAC is not just a passive regulator. When institutions fall short, the consequences are severe and public.
The Crown Resorts Case
In 2022, Crown Melbourne and Crown Perth were found guilty of systemic AML/CTF program failures. AUSTRAC investigations revealed:
- Inadequate risk assessments of high-risk customers and junket operators
- Poor transaction monitoring
- Weak governance and oversight
Penalty: AUD 450 million settlement
Impact: Major reputational damage and licence scrutiny
The Westpac Case
Arguably, the most consequential case in Australia’s AML history. In 2020, Westpac was fined AUD 1.3 billion — the largest civil penalty in Australian corporate history — for:
- Failing to report over 23 million IFTIs
- Inadequate transaction monitoring
- Enabling transactions linked to child exploitation networks
These cases underscore the high expectations placed on financial institutions — not just to comply, but to detect, investigate, and prevent abuse of their services.
Australia’s AML Pain Points and What Tranche 2 Means
Unregulated Professions: The Tranche 2 Gap
Australia’s AML/CTF regime currently does not cover “gatekeeper” professions — lawyers, accountants, real estate agents, and company service providers. This gap has drawn criticism from both the FATF and domestic watchdogs.
Tranche 2, expected to be legislated in 2025, will:
- Extend AML obligations to these sectors
- Close critical vulnerabilities exploited for shell companies, illicit property purchases, and tax evasion
- Align Australia with global AML standards
For fintechs and financial institutions, this will mean greater scrutiny of third-party relationships and new customer categories.
FATF Evaluation: Australia Under the Global Lens
The Financial Action Task Force (FATF) — the global AML watchdog — is expected to conduct its next mutual evaluation of Australia soon. In its last review, Australia was flagged for:
- Delays in enacting Tranche 2 reforms
- Over-reliance on self-regulation in some sectors
- Inconsistent enforcement levels
AUSTRAC and the government are now under pressure to demonstrate tangible improvements, including:
- Broader coverage of at-risk sectors
- Better risk-based supervision
- More tech-led compliance outcomes
How Fintechs Can Stay Ahead
For fintechs, the AML/CTF journey can seem overwhelming, especially when scaling across regions. Here are five key steps to staying ahead:
- Invest Early in AML Infrastructure
Don’t wait until licensing or audits to build compliance controls. - Use Technology to Monitor in Real-Time
Especially for high-velocity, small-value transactions common in wallets or P2P services. - Customise Risk Scoring
A high-risk customer in lending may not be the same as one in gaming or cross-border remittances. - Build for Scalability
Choose AML platforms that can grow with you, not patchwork solutions. - Stay Informed on Regional Variations
AUSTRAC’s expectations differ from MAS (Singapore) or BSP (Philippines); know your market.
Why AML Tech Is No Longer Optional
In today’s landscape, manual reviews and static rules don’t cut it. Criminals move faster — and so must compliance teams.
Key advantages of modern AML platforms:
- Machine learning-based transaction monitoring
- Dynamic threshold calibration to reduce false positives
- Real-time alerting and case triage
- Behavioural profiling and pattern recognition
- Audit-ready investigation trails
How Tookitaki Helps You Stay Ahead
Tookitaki’s FinCense platform is purpose-built to tackle the real challenges banks and fintechs face in Australia and across APAC.
Key Modules:
🔹 Customer Onboarding Suite
Seamlessly integrates KYC, risk profiling, and watchlist screening
🔹 Transaction Monitoring
Scenario-based detection using patterns from the AFC Ecosystem
🔹 Smart Screening
Covers national ID, aliases, and local nuances — built to minimise false positives
🔹 FinMate (AI Copilot)
Assists investigators with summarised case narratives, red flags, and recommendations
Collaborative Advantage:
FinCense is powered by the AFC Ecosystem — a global community where financial institutions share typologies and red flags anonymously. This collective intelligence improves detection and reduces blind spots for all members.
For institutions facing rising risks from cross-border scams, shell company abuse, and real-time laundering, Tookitaki offers a smarter, community-driven alternative to traditional rule engines.
%2520(1).webp)
Final Thoughts: A Smarter Future Starts Now
AUSTRAC’s expanding role and the upcoming Tranche 2 reforms signal a future where compliance will be more inclusive, tech-powered, and intelligence-driven.
For banks and fintechs, the opportunity lies not just in complying, but in leading. With the right tools, collaborative frameworks, and forward-thinking partners like Tookitaki, staying ahead of both regulation and risk is no longer an aspiration — it’s an expectation.

The Thai Investment Scam That Sold Dreams and Stole Millions from Australians
In an era where trust is everything, a well-orchestrated investment scam has shaken thousands of unsuspecting Australians—reminding us that financial fraud is becoming smarter, faster, and more global.
In June 2025, Thai police arrested 13 foreign nationals operating a fake investment scheme that swindled over 14,000 Australians, totalling USD 1.2 million in losses. The arrests marked a breakthrough, but also exposed a growing web of cross-border fraud built on digital deception.
Background of the Scam
How the Scam Worked
The fraudsters posed as investment consultants from legitimate-sounding financial firms. Using spoofed Australian phone numbers, they cold-called thousands of individuals across the country and offered attractive bond investment opportunities. These pitches came with fake documentation, official logos, and scripted professionalism designed to build trust quickly.
Key elements of the modus operandi:
- Cold Calls: Made using VoIP services that masked the true origin.
- Fake Bonds: Named similarly to well-known offerings like “Liberty Bonds”.
- Pressure Tactics: Victims were told the opportunity was limited-time and required urgent action.
- Credibility Builders: They shared fraudulent certificates, fake websites, and even customer service follow-ups.
The syndicate used psychological manipulation and urgency to override rational scepticism—a classic hallmark of modern investment fraud.

What the Case Revealed
The arrests revealed a shocking level of operational maturity:
- The scam was run like a call centre—with job roles, scripts, and tech infrastructure.
- Laptops, phones, documents, and hard drives found on-site pointed to thousands of victim profiles.
- The scammers were not Thai nationals—they were foreigners recruited for their English skills and familiarity with Australian culture.
This case also confirmed suspicions that Southeast Asia is increasingly a base of operations for globally targeted scams due to loosely regulated digital infrastructure and low operational costs.

Impact on Global Finance
The Thai investment scam isn’t an isolated financial crime—it’s a sign of larger systemic risks:
🌐 Cross-Border Vulnerabilities
- Victims in Australia, syndicates in Thailand, digital payments routed through intermediary countries—a truly borderless operation.
- This highlights weaknesses in international anti-fraud collaboration, especially in early detection and enforcement.
📉 Institutional Trust Erosion
- Thousands of Australians now second-guess legitimate investment outreach.
- As frauds mimic real financial products, trust in banks, fintechs, and brokers is undermined—especially among older investors.
💸 Rising Compliance Costs
- Financial institutions face pressure to tighten onboarding, verification, and investment approval protocols.
- These measures, while necessary, increase costs and slow down legitimate operations.
🔁 Abuse of Financial Infrastructure
- Scammers moved stolen funds via mule accounts, prepaid cards, and potentially crypto wallets, complicating recovery efforts and aiding money laundering.
This scam may be small in dollar terms compared to global Ponzi schemes, but in structural impact, it’s significant.
Lessons Learned from the Scam
The Thai scam case offers valuable lessons for all financial stakeholders:
1. Scams are Scalable
Fraud is no longer amateur—it’s an industrialised business model with SOPs, tech stacks, and recruitment funnels. Treat it like a business, not a one-off incident.
2. Consumer Awareness is a Weak Link
Even financially literate individuals were duped. The emotional triggers and sophisticated documentation overpowered rational caution. Public education must be ongoing and adaptive.
3. Older Demographics Need Targeted Protection
A large portion of the victims were retirees or older professionals—an age group with access to capital but less digital scam awareness.
4. Transnational Law Enforcement Is Critical
The success of this bust hinged on coordination between Thai police and Australian authorities. Stronger intelligence-sharing, extradition treaties, and regional enforcement frameworks are now vital.
5. Scams Are Brand Killers
Reputational damage from such scams doesn’t just affect the victims—it casts a shadow on entire categories of legitimate financial products.
The Role of Technology in Preventing Future Scandals
As scams grow more sophisticated, traditional rule-based compliance systems are no longer enough. Financial institutions must move towards intelligent, adaptive, and collaborative technologies.
1. AI-Powered Transaction Monitoring
Detecting subtle anomalies—such as unusually timed investments, repeat transactions to flagged accounts, or first-time investors sending large sums—requires machine learning models that learn and adapt from real-time data.
2. Collaborative Intelligence
Scams often follow repeated patterns. A federated approach allows institutions to share risk indicators and red flags without exposing customer data—building collective muscle to fight new threats faster.
3. Behavioural Risk Modelling
Beyond static thresholds, systems can now track behavioural shifts—like a customer suddenly engaging in high-risk investments, or funds moving through unfamiliar geographies.
4. Continuous Learning
Fraud is dynamic—your defences must be too. Tools that ingest new typologies, simulate red flag thresholds, and auto-tune detection parameters are the future of scalable protection.
Moving Forward: Learning from the Past, Preparing for the Future
This scam underscores the urgent need for proactive, intelligence-led financial crime defence strategies. Institutions can no longer afford to act alone, react late, or rely solely on static rules.
This is where Tookitaki’s FinCense platform comes in. Purpose-built for the new era of fraud and compliance, it enables:
- 🔍 Advanced typology-based detection that spots patterns in transactions, behaviour, and cross-border flow.
- 🤖 Federated learning models that update with every new red flag scenario contributed by a global expert community.
- 🛡️ Scenario simulation engines to test your institution’s resilience to evolving scams before they strike.
- 📉 Smart dispositioning to reduce false positives while capturing real threats early.
Tookitaki’s platform, powered by the AFC Ecosystem, transforms compliance teams from passive detectors into active defenders.
%2520(1).webp)
Conclusion
The Thai investment fraud case was more than a scam—it was a systems test. A test of our global defences, our public awareness, and our institutional resilience.
Thousands lost money. But the real loss would be if we ignored what this case revealed.
It’s time for the financial ecosystem to level up—combining technology, collaboration, and foresight to stay ahead of an increasingly professional fraud economy.
Because the next scam is already being planned.
The question is: will we be ready?

Australia on Alert: Why Financial Crime Prevention Needs a Smarter Playbook
From traditional banks to rising fintechs, Australia's financial sector is under siege—not from market volatility, but from the surging tide of financial crime. In recent years, the country has become a hotspot for tech-enabled fraud and cross-border money laundering.
A surge in scams, evolving typologies, and increasingly sophisticated actors are pressuring institutions to confront a hard truth: the current playbook is outdated. With fraudsters exploiting digital platforms and faster payments, financial institutions must now pivot from reactive defences to real-time, intelligence-led prevention strategies.
The Australian government has stepped up through initiatives like the National Anti-Scam Centre and legislative reforms—but the real battleground lies inside financial institutions. Their ability to adapt fast, collaborate widely, and think smarter will define who stays ahead.
{{cta-first}}
The Evolving Threat Landscape
Australia’s shift to instant payments via the New Payments Platform (NPP) has revolutionised financial convenience. However, it's also reduced the window for detecting fraud to mere seconds—exposing institutions to high-velocity, low-footprint crime.
In 2024, Australians lost over AUD 2 billion to scams, according to the ACCC’s Scamwatch report:
- Investment scams accounted for the largest losses at AUD 945 million
- Remote access scams followed with AUD 106 million
- Other high-loss categories included payment redirection and phishing scams
Behind many of these frauds are organised crime groups that exploit vulnerabilities in onboarding systems, mule account networks, and compliance delays. These syndicates operate internationally, often laundering funds through unsuspecting victims or digital assets.
Recent alerts from AUSTRAC and ASIC also highlighted the misuse of cryptocurrency exchanges, online gaming wallets, and e-commerce platforms in money laundering schemes. The message is clear: financial crime is mutating faster than most defences can adapt.
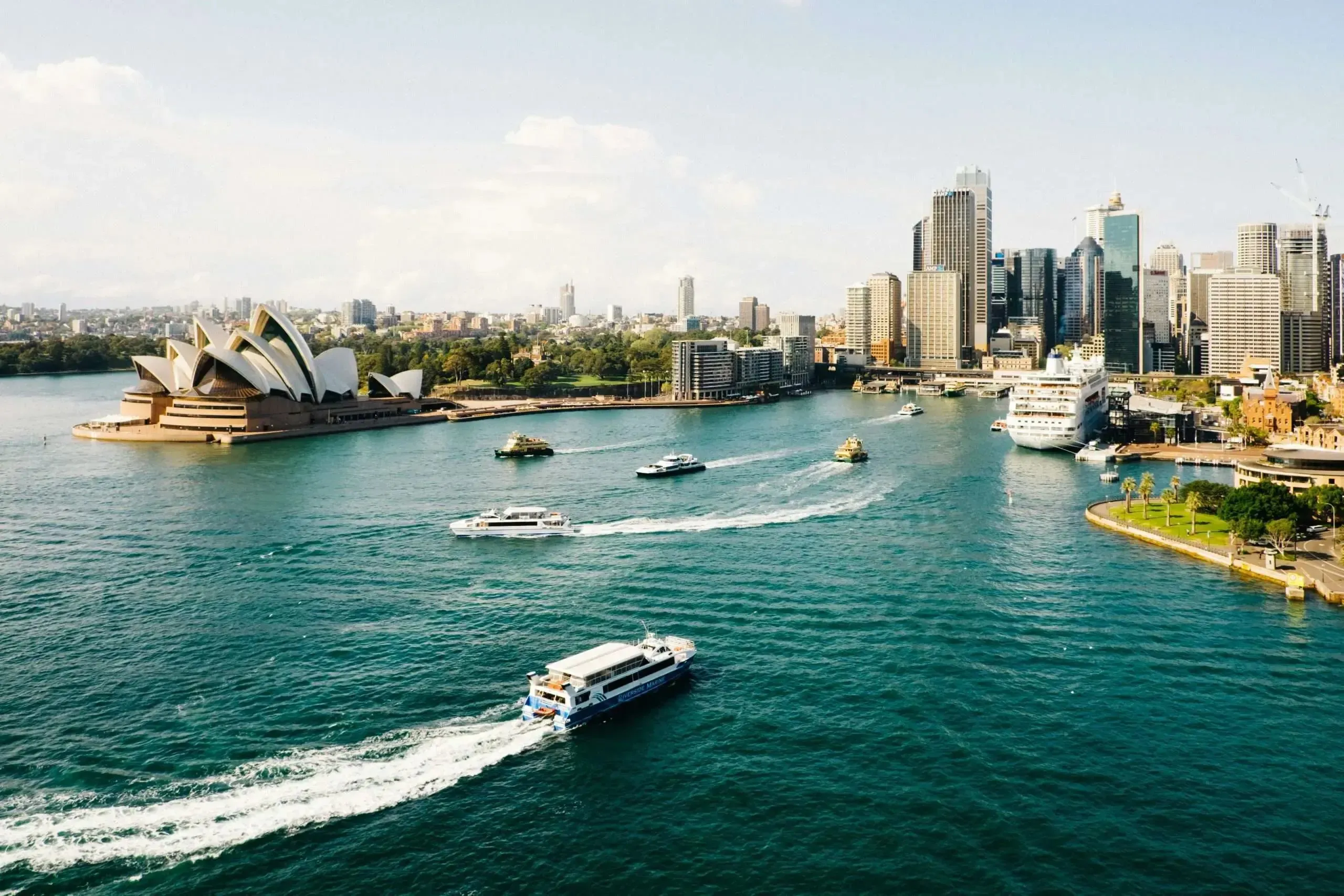
Why Traditional Defences Are Falling Short
Despite growing threats, many financial institutions still rely on legacy systems that were designed for a static risk environment. These tools:
- Depend on manual rule updates, which can take weeks or months to deploy
- Trigger false positives at scale, overwhelming compliance teams
- Operate in silos, with no shared visibility across institutions
For instance, a suspicious pattern flagged at one bank may go entirely undetected at another—simply because they don’t share learnings. This fragmented model gives criminals a huge advantage, allowing them to exploit gaps in coverage and coordination.
The consequences aren’t just operational—they’re strategic. As financial criminals embrace automation, phishing kits, and AI-generated deepfakes, institutions using static tools are increasingly being outpaced.
The Cost of Inaction
The financial and reputational fallout from poor detection systems can be severe.
1. Consumer Trust Erosion
Australians are increasingly vocal about scam experiences. Victims often turn to social media or regulators after being defrauded—especially if they feel the bank was slow to react or dismissive of their case.
2. Regulatory Enforcement
AUSTRAC has made headlines with its tough stance on non-compliance. High-profile penalties against Crown Resorts, Star Entertainment, and non-bank remittance services show that even giants are not immune to scrutiny.
3. Market Reputation Risk
Investors and partners view AML and fraud management as core risk factors. A single failure can trigger media attention, customer churn, and long-term brand damage.
The bottom line? Institutions can no longer afford to treat compliance as a cost centre. It’s a driver of brand trust and operational resilience.
Rethinking AML and Fraud Prevention in Australia
As criminal innovation continues to escalate, the defence strategy must be proactive, intelligent, and collaborative. The foundations of this smarter approach include:
✅ AI-Powered Detection Systems
These systems move beyond rule-based alerts to analyse behavioural patterns in real-time. By learning from past frauds and adapting dynamically, AI models can flag suspicious activity before it becomes systemic.
For example:
- Unusual login behaviour combined with high-value NPP transfers
- Layered payments through multiple prepaid cards and wallets
- Transactions just under the reporting threshold from new accounts
These patterns may look innocuous in isolation, but form high-risk signals when viewed in context.
✅ Federated Intelligence Sharing
Australia’s siloed infrastructure has long limited inter-institutional learning. A federated model enables institutions to share insights without exposing sensitive data—helping detect emerging scams faster.
Shared typologies, red flags, and network patterns allow compliance teams to benefit from collective intelligence rather than fighting crime alone.
✅ Human-in-the-Loop Collaboration
Technology is only part of the answer. AI tools must be designed to empower investigators, not replace them. When AI surfaces the right alerts, compliance professionals can:
- Reduce time-to-investigation
- Make informed, contextual decisions
- Focus on complex cases with real impact
This fusion of human judgement and machine precision is key to staying agile and accurate.
A Smarter Playbook in Action: How Tookitaki Helps
At Tookitaki, we’ve built an ecosystem that reflects this smarter, modern approach.
FinCense is an AI-native platform designed for real-time detection across fraud and AML. It automates threshold tuning, uses network analytics to detect mule activity, and continuously evolves with new typologies.
The AFC Ecosystem is our collaborative network of compliance professionals and institutions who contribute real-world risk scenarios and emerging fraud patterns. These scenarios are curated, validated, and available out-of-the-box for immediate deployment in FinCense.
Some examples already relevant to Australian institutions include:
- QR code-enabled scams using fake invoice payments
- Micro-laundering via e-wallet top-ups and fast NPP withdrawals
- Cross-border layering involving crypto exchanges and shell businesses
Together, FinCense and the AFC Ecosystem enable institutions to:
- Detect faster
- Collaborate smarter
- Reduce false positives
- Stay regulator-ready
Building a Future-Ready Framework
The question is no longer if financial crime will strike—it’s how well prepared your institution is when it does.
To be future-ready, institutions must:
- Break silos through collaborative platforms
- Invest in continuous learning systems that evolve with threats
- Equip teams with intelligent tools, not more manual work
Those who act now will not only improve operational resilience, but also lead in restoring public trust.
As the financial landscape transforms, so too must the compliance infrastructure. Tomorrow’s threats demand a shared response, built on intelligence, speed, and community-led innovation.
%2520(1).webp)
Conclusion: Trust Is the New Currency
Australia is at a turning point. The cost of reactive, siloed compliance is too high—and criminals are already exploiting the lag.
It’s time to adopt a smarter playbook. One where technology, collaboration, and shared intelligence replace outdated controls.
At Tookitaki, we’re proud to build the Trust Layer for Financial Services—empowering banks and fintechs to:
- Stop fraud before it escalates
- Reduce false positives and compliance fatigue
- Strengthen transparency and accountability
Through FinCense and the AFC Ecosystem, our mission is simple: enable smarter decisions, faster actions, and safer financial systems.
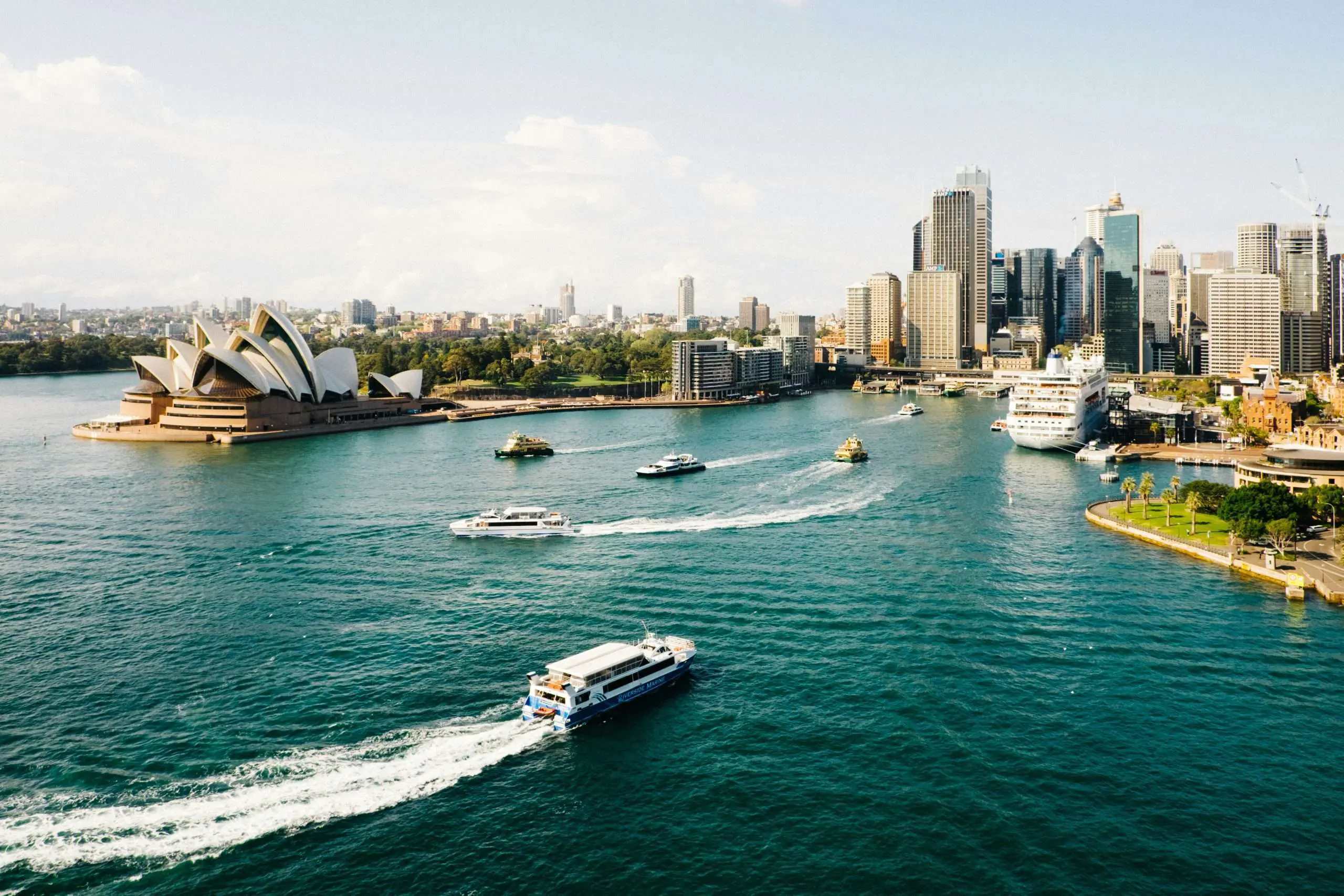
Behind the Compliance Curtain: The Future of AML in Australia
Australia’s sunny financial reputation has come under scrutiny—and this time, the spotlight is global.
From casino scandals to multi-billion-dollar remittance breaches, the country’s anti-money laundering (AML) framework is facing a pivotal moment. What was once seen as a gold standard in regional governance is now under pressure to catch up—and compliance officers across banks, fintechs, and regulatory bodies are watching closely.
So what lies behind the curtain of AML in Australia today—and what must the financial community do next?

The AML Landscape in Australia: Where Things Stand
Australia’s AML/CFT regime has long been led by AUSTRAC, the nation’s financial intelligence unit and regulator. Over the past few years, AUSTRAC has made headlines with major enforcement actions:
- Westpac (2020): A $1.3 billion penalty over 23 million breaches of AML laws.
- Crown Resorts (2022): Systemic failure to monitor high-risk transactions, especially tied to junket operators and casinos.
- Star Entertainment Group (2022): Similar failings in AML controls and customer due diligence.
These cases revealed a troubling pattern: AML risks were known, red flags existed, but institutions lacked either the technology, urgency, or capability to respond in real time.
More worryingly, Australia’s AML legal framework—particularly its coverage of non-financial sectors like lawyers, accountants, real estate agents, and high-value dealers—remains incomplete. This gap in regulatory coverage continues to raise red flags with global watchdogs, especially the Financial Action Task Force (FATF).
The Tranche 2 Reforms: Closing the Gaps or Buying Time?
For nearly two decades, Australia has delayed implementing the so-called Tranche 2 reforms, which would bring designated non-financial businesses and professions (DNFBPs) into the AML regulatory net.
What Tranche 2 Proposes:
- AML obligations for real estate professionals, lawyers, accountants, and company service providers.
- Stronger beneficial ownership transparency.
- Enhanced customer due diligence and reporting mechanisms across non-financial channels.
Yet, while successive governments have pledged action, progress has been sluggish. Industry bodies have raised concerns about cost, feasibility, and regulatory overreach. But international momentum is building, and patience is wearing thin.
In its 2023 follow-up review, FATF explicitly called out Australia’s delayed reforms. Without Tranche 2, the country faces increased scrutiny—and potential reputational damage that could affect correspondent banking relationships and investor trust.

The Tech Factor: How Modern AML Looks in 2025
Even where regulations exist, legacy compliance systems are struggling to keep up with today’s threats. Financial crime has evolved. So must the tools to fight it.
What’s Changed:
- Speed: Real-time payments and digital wallets mean funds can be layered, split, and moved across jurisdictions in seconds.
- Complexity: Fraudsters are using mules, shell companies, and social engineering to blend illicit flows with legitimate ones.
- Volume: Transaction volumes are rising, making manual reviews and static rules increasingly unviable.
Modern AML compliance now demands real-time monitoring, behavioural analysis, and AI-driven detection engines that adapt to new patterns as they emerge. This is where advanced platforms like Tookitaki’s FinCense come in—offering scenario-driven intelligence and federated learning capabilities tailored for high-risk markets like Australia.
Case Insight: Where Detection Failed—and Where Tech Could Have Helped
Consider the AUSTRAC case against Crown Resorts. Red flags—such as large, unexplained cash deposits, transactions linked to politically exposed persons (PEPs), and high-risk jurisdictions—were not acted upon for months, sometimes years.
The problem wasn’t a lack of data. It was a failure to connect the dots in real time.
With an adaptive AML system like FinCense in place, the scenario might have looked different:
- Suspicious transaction patterns would have triggered real-time alerts.
- Beneficiary risk scoring could have flagged high-risk links earlier.
- AI-based learning could have surfaced anomalous activity invisible to static rule sets.
The outcome? Faster intervention, reduced institutional risk, and regulatory confidence.
Building the Future: Tookitaki’s Role in Strengthening Australia’s AML Defences
Tookitaki’s FinCense platform is designed for the complexity of modern financial ecosystems—especially those navigating regulatory reform and reputational pressure, like Australia.
Key Features That Matter:
- Federated Learning Engine: Enables institutions to learn from emerging typologies across the region—without sharing sensitive data.
- Real-Time Transaction Monitoring: Uses AI to surface anomalous patterns and risk indicators at the speed of today’s financial crime.
- Scenario-Based Approach: Combines regulatory intelligence with real-world cases to keep detection capabilities relevant and context-rich.
- Audit-Ready Investigations: Helps compliance teams manage alerts, document findings, and demonstrate control effectiveness.
As Tranche 2 looms and regulatory expectations rise, FinCense can help banks and fintechs in Australia stay ahead of both criminal innovation and regulatory demand.
What Compliance Teams Must Do Now
✅ Prepare for Tranche 2 (Even If It’s Not Here Yet)
- Map exposure to DNFBPs.
- Engage with vendors and consultants to scope out necessary controls.
✅ Build for Agility and Resilience
- Invest in dynamic risk-scoring engines and AI-powered analytics.
- Integrate systems that can adapt, not just flag transactions.
✅ Collaborate and Learn
- Participate in intelligence-sharing platforms like the AFC Ecosystem.
- Use scenario libraries to anticipate typologies before they strike.
✅ Rethink ROI from an AML Lens
- With regulators now tracking the effectiveness (not just existence) of AML systems, demonstrate real-time capability, reduced false positives, and improved investigation turnaround.
%2520(1).webp)
Conclusion: The Curtain’s Up—What Will Australia Do Next?
Australia stands at a crossroads. Behind the curtain of its legacy AML system lies both risk and opportunity.
The risk is clear: continued global scrutiny, regulatory gaps, and potential grey listing if reforms stall.
But the opportunity is greater: to lead the region with tech-driven, intelligence-led compliance that’s faster, smarter, and more collaborative than ever.
As the regulatory environment evolves, so must the institutions within it. With the right partners, like Tookitaki, and a commitment to real-time defences, Australia can transform its AML posture from reactive to revolutionary.
Because in the fight against financial crime, detection is no longer enough. It’s time to defend.

Inside AUSTRAC: Navigating Australia’s AML/CTF Regulations in a High-Risk Era
As money laundering methods grow more sophisticated, the pressure on financial institutions to detect, report, and prevent financial crime is intensifying — and AUSTRAC is at the centre of it all.
In an era where financial ecosystems are rapidly digitising, AUSTRAC’s role in overseeing Anti-Money Laundering (AML) and Counter-Terrorism Financing (CTF) compliance has become mission-critical. For banks, fintechs, and other reporting entities, staying ahead of regulatory expectations is no longer just a compliance issue — it’s a matter of reputation, trust, and long-term viability.
In this blog, we explore:
- AUSTRAC’s mandate and structure
- Key AML/CTF obligations under Australian law
- Landmark enforcement cases
- Upcoming reforms, including Tranche 2
- FATF scrutiny and global compliance pressures
- How tech-forward compliance strategies are reshaping the future

What is AUSTRAC and Why Does It Matter?
AUSTRAC — the Australian Transaction Reports and Analysis Centre — is the government body responsible for detecting and disrupting criminal abuse of Australia’s financial system.
AUSTRAC has a dual mandate:
- Regulator: Supervises compliance with AML/CTF obligations.
- Financial Intelligence Unit (FIU): Collects and analyses data to support law enforcement, national security, and international counterparts.
It works with over 17,000 reporting entities, ranging from traditional banks to digital wallets, remittance providers, gaming platforms, and more. As both a data collector and enforcer, AUSTRAC is uniquely positioned to uncover illicit financial activity at scale.
A Brief History of AML/CTF Regulation in Australia
Australia’s journey in strengthening its anti-money laundering and counter-terrorism financing framework began in earnest with the passage of the AML/CTF Act in 2006. This legislation introduced foundational obligations such as KYC procedures, transaction monitoring, and reporting requirements for a wide range of financial institutions and service providers.
Over time, the regime has evolved significantly. In 2014, AUSTRAC formalised the risk-based approach, requiring entities to tailor their AML programs based on their specific exposure to financial crime risks.
The period between 2018 and 2020 marked a turning point in enforcement, with AUSTRAC taking decisive action against some of Australia’s largest institutions — including Tabcorp, the Commonwealth Bank, and Westpac — for major compliance failures.
In the years that followed, Tranche 2 reforms were proposed to expand AML/CTF obligations to include professions such as lawyers, accountants, and real estate agents, which are known to be exploited for laundering illicit funds.
As of 2024, these reforms remain under active discussion, with the Australian government under growing pressure from international bodies such as the FATF to close regulatory gaps. The expected passage of Tranche 2 in 2025 would significantly broaden AUSTRAC’s regulatory reach and bring Australia closer in line with global AML standards.

Understanding Your AML/CTF Obligations
If your institution provides “designated services” under the AML/CTF Act, here’s what you’re required to do:
🔹 AML/CTF Program (Part A and Part B)
- Part A: Institutional risk assessments, governance, reporting, and training
- Part B: Customer identification and verification procedures (KYC)
🔹 Reporting Requirements
- Suspicious Matter Reports (SMRs)
Must be submitted when the activity raises suspicion, regardless of the amount. - Threshold Transaction Reports (TTRs)
For cash transactions of AUD 10,000 or more. - International Funds Transfer Instructions (IFTIs)
Mandatory for cross-border fund movements.
🔹 Customer Due Diligence (CDD)
- Verify customer identity at onboarding
- Apply Enhanced Due Diligence (EDD) for high-risk customers or transactions
- Conduct ongoing monitoring
🔹 Record Keeping
- Maintain transaction and identity verification records for at least 7 years.
AUSTRAC’s Enforcement Power: Learning from Past Failures
AUSTRAC is not just a passive regulator. When institutions fall short, the consequences are severe and public.
The Crown Resorts Case
In 2022, Crown Melbourne and Crown Perth were found guilty of systemic AML/CTF program failures. AUSTRAC investigations revealed:
- Inadequate risk assessments of high-risk customers and junket operators
- Poor transaction monitoring
- Weak governance and oversight
Penalty: AUD 450 million settlement
Impact: Major reputational damage and licence scrutiny
The Westpac Case
Arguably, the most consequential case in Australia’s AML history. In 2020, Westpac was fined AUD 1.3 billion — the largest civil penalty in Australian corporate history — for:
- Failing to report over 23 million IFTIs
- Inadequate transaction monitoring
- Enabling transactions linked to child exploitation networks
These cases underscore the high expectations placed on financial institutions — not just to comply, but to detect, investigate, and prevent abuse of their services.
Australia’s AML Pain Points and What Tranche 2 Means
Unregulated Professions: The Tranche 2 Gap
Australia’s AML/CTF regime currently does not cover “gatekeeper” professions — lawyers, accountants, real estate agents, and company service providers. This gap has drawn criticism from both the FATF and domestic watchdogs.
Tranche 2, expected to be legislated in 2025, will:
- Extend AML obligations to these sectors
- Close critical vulnerabilities exploited for shell companies, illicit property purchases, and tax evasion
- Align Australia with global AML standards
For fintechs and financial institutions, this will mean greater scrutiny of third-party relationships and new customer categories.
FATF Evaluation: Australia Under the Global Lens
The Financial Action Task Force (FATF) — the global AML watchdog — is expected to conduct its next mutual evaluation of Australia soon. In its last review, Australia was flagged for:
- Delays in enacting Tranche 2 reforms
- Over-reliance on self-regulation in some sectors
- Inconsistent enforcement levels
AUSTRAC and the government are now under pressure to demonstrate tangible improvements, including:
- Broader coverage of at-risk sectors
- Better risk-based supervision
- More tech-led compliance outcomes
How Fintechs Can Stay Ahead
For fintechs, the AML/CTF journey can seem overwhelming, especially when scaling across regions. Here are five key steps to staying ahead:
- Invest Early in AML Infrastructure
Don’t wait until licensing or audits to build compliance controls. - Use Technology to Monitor in Real-Time
Especially for high-velocity, small-value transactions common in wallets or P2P services. - Customise Risk Scoring
A high-risk customer in lending may not be the same as one in gaming or cross-border remittances. - Build for Scalability
Choose AML platforms that can grow with you, not patchwork solutions. - Stay Informed on Regional Variations
AUSTRAC’s expectations differ from MAS (Singapore) or BSP (Philippines); know your market.
Why AML Tech Is No Longer Optional
In today’s landscape, manual reviews and static rules don’t cut it. Criminals move faster — and so must compliance teams.
Key advantages of modern AML platforms:
- Machine learning-based transaction monitoring
- Dynamic threshold calibration to reduce false positives
- Real-time alerting and case triage
- Behavioural profiling and pattern recognition
- Audit-ready investigation trails
How Tookitaki Helps You Stay Ahead
Tookitaki’s FinCense platform is purpose-built to tackle the real challenges banks and fintechs face in Australia and across APAC.
Key Modules:
🔹 Customer Onboarding Suite
Seamlessly integrates KYC, risk profiling, and watchlist screening
🔹 Transaction Monitoring
Scenario-based detection using patterns from the AFC Ecosystem
🔹 Smart Screening
Covers national ID, aliases, and local nuances — built to minimise false positives
🔹 FinMate (AI Copilot)
Assists investigators with summarised case narratives, red flags, and recommendations
Collaborative Advantage:
FinCense is powered by the AFC Ecosystem — a global community where financial institutions share typologies and red flags anonymously. This collective intelligence improves detection and reduces blind spots for all members.
For institutions facing rising risks from cross-border scams, shell company abuse, and real-time laundering, Tookitaki offers a smarter, community-driven alternative to traditional rule engines.
%2520(1).webp)
Final Thoughts: A Smarter Future Starts Now
AUSTRAC’s expanding role and the upcoming Tranche 2 reforms signal a future where compliance will be more inclusive, tech-powered, and intelligence-driven.
For banks and fintechs, the opportunity lies not just in complying, but in leading. With the right tools, collaborative frameworks, and forward-thinking partners like Tookitaki, staying ahead of both regulation and risk is no longer an aspiration — it’s an expectation.
