Roundtable Rewind: Embracing Next-gen Tech & Collaboration
As a regtech player with a vision to enable sustainable compliance programs in financial institutions, Tookitaki organized a first-of-its-kind industry roundtable in Charlotte, NC, USA to discuss opportunities to innovate current AML programs and meet regulatory expectations. The roundtable was an open forum for various stakeholder groups to ideate on how to assimilate new-edge technologies like artificial intelligence (AI) and machine learning (ML) within their system of internal controls and across the industry to improve the detection and investigation of illicit financial flows.
{{ cta-first }}
It saw participation from global financial institutions, risk and compliance professionals, consulting firms and technology services firms. Long time AML industry veteran and associate director at Protiviti, George Harris, moderated the discussion topics. The roundtable addressed the following questions:
• How can we create a sustainable AML program design and address challenges associated with today’s compliance processes and systems?
• What role can modern technology play in bringing together banks’ money laundering wisdom?
• How can we deploy an AI model in production with scale and mitigate compliance risk?
Sustainability and modern tech
Our participants unanimously agreed that the general skepticism surrounding the adoption of AI and ML to address regulatory compliance issues has started fading away as such technologies meet applicable security guidelines, improves overall operational performance, and improves customer experience. However, the industry experts suggested specific key points, while adopting modern technologies to create sustainable compliance programs.
1. Behavior monitoring rather than transactional monitoring is the need of the hour.
As threshold setting may not be always effective to identify suspicious transactions, it’s imperative on today’s compliance programs to follow a behavior monitoring approach. They need to understand what normal behavior is to find out whether specific cases standout from that normal behavior. If we can identify the normal behavior, then we can weed out those non-productive cases much faster and in a sustainable way. To understand customers’ true banking behavior, we need to go beyond pre-defined banking segments and transactions and look at them from a holistic point of view. This approach would bring together all relevant customer information such as lifetime STRs/SARs, multiple accounts, demographics, and relationship with counterparties to create customer groups and identify normal and abnormal behavior.
2. Compliance programs are not sustainable unless they are explainable. Transparency has been an issue with AI/ML-powered solutions in any area. RegTech solutions are no exception. Results from a compliance solution should be explainable and auditable so that there is a complete audit trail to back up whatever decision a compliance professional is taking to handle all non-productive cases.
3. Data integrity is at the heart of machine learning.
To unlock the full potential of machine learning in compliance programs, the quality of input data must be verified. The first step in generating quality output from a machine learning model is to ensure that the right quality data is going into the model. If things are really bad, machine learning is nothing but garbage.
4. Less sophistication is better.
One of the problems with modern technologies such as Big Data and AI is that they require highly specialized, expensive people. While looking for AI-powered AML software, ensure it offers explanations for its output in business-friendly language, easy to implement with existing banking infrastructure and low in maintenance.
5. Secondary scoring is a nice way to transition to a new paradigm.
Though basic systems can get “incrementally matter, they can’t become materially better”. At some point in time, the industry is going to recognize, particularly regulators, that innovation is to come and take over. Machine learning is taking over our lives in multi-faceted ways. When we apply machine learning to financial crimes, the fundamental question is how we will move towards the new technology without disrupting the normal business flow and how we will do that in a low-risk way. Secondary scoring is a nice way to transition compliance to a new paradigm. If we layer in machine learning over whatever technologies currently in use, then over time, banks can shift towards machine learning models and phase out the older technology without disrupting business. Subsequently, you may put in an infrastructure of analytics that will serve the whole lifecycle of the behavioral process, onboarding with name matching, transaction monitoring, and investigations.
Collaboration and robust compliance programs
Banks are always looking to detect non-productive alerts in a better way so that they can prioritize alerts better. Currently, they are building rules or machine learning models on top of rules in silos to solve compliance issues. These rules and models are built based on information available to individual banks. However, if we have to move to the next generation of compliance systems, we need to go beyond these custom models that we are building. The fundamental challenge we have is building an ecosystem where each bank can share money laundering patterns to create a robust library of patterns that can help banks make their models more powerful in terms of detecting those scenarios beyond what they already have.
However, as banks cannot share their customer data, building a pattern sharing AML ecosystem is a real challenge. Tookitaki has brought in innovation to address the challenge and offer financial institutions an ability to share money laundering patterns, without data leakage. The approach helps banks enrich their detection rate for suspicious cases, especially complex structuring cases, with significantly low misclassification rates. There had been questions on the practicality of the novel concept. The most important ones and their responses are noted down below.
1. Is pattern sharing applicable across different types of financial institutions?
It’s up to the banks to decide whether they want a specific pattern or not. Also, the typologies work on each banks’ data. If the scenarios are not applicable to your data, then they will not be effective. As part of the regulatory approval, it has to be justified that the framework works or not. For that, it has to undergo rigorous testing, including under-the-line testing and over-the-line testing, and the results are to be validated at the segment level and the rules level.
2. Will the pattern-sharing framework replace existing solutions?
Most of Tookitaki’s regional and global deployments have been complementary solutions. We are looking at providing incremental results by adding new typologies without a ‘rip and replace’. Tookitaki solution can sit on top of existing solutions and drastically improve alerts prioritization with a 50% reduction in false positives. That level of efficiency can only happen when we can bring in industry knowledge rather than siloed knowledge.
3. Will it make sense if there is regulatory pressure to continuously update models?
By default, the concept follows a champion-challenger framework, where the challenger model needs to undergo various validation steps to become a champion model. While regulations for AI-powered model governance in the financial services industry are yet to mature, there are guidelines that banks can follow while taking up AI. Singapore’s FEAT framework (fairness, ethics, accountability, and transparency) and the US OCC guidance on model risk management can be used to promote the ethical and unbiased use of AI within financial institutions.
{{cta-guide}}
Pursuing the AI Journey for AML
Moving from innovation experimentation to day-to-day enterprise usage requires engineering excellence and a business case for change. It’s often more than ROI that banks consider while adopting a fresh approach to AML risk management.
1. It is important to get examiners to agree with you.
As standard setters in the industry, examiners play a crucial role in the adoption of AI in financial services. As one of the initial steps, it is recommended that business stakeholders talk to examiners, demo the solution and get their buy-in. Various examiners compete with each other, so if we can get one to adopt modern technology, the others will follow.
2. A proper balance between better risk management and monetary benefits is crucial.
While ROI is important for each and every bank, the benefits from the use of modern technology in regulatory compliance are primarily about augmented risk management and the ability to do compliance jobs better. These will obviously result in financial benefits in the long run in addition to the competitive edge over peers.
3. Have we reached a machine vs. human state? Not as of now.
As of now, we cannot take humans out of the compliance process and replace them with machine learning. Human intuition is something that cannot be replaced. Modern compliance technology looks to assist human beings with better analytics and intelligence so that they can do their work faster and better.
Conclusion
The roundtable concluded that the future of regulatory compliance will be powered by advanced technology. As criminals are using technology to outsmart legacy systems, financial institutions are lagging behind with process inefficiencies and heightened risk. The use of right technology can help them mitigate risk as well as reduce operational costs significantly. It is important to create awareness about novel technological approaches in the market to address AML compliance and ensure sustainability. In addition, collaboration among various industry stakeholders such as financial institutions, regulators and technology firms can surely alter the current AML compliance picture and make the world a better place to live.
Experience the most intelligent AML and fraud prevention platform
Experience the most intelligent AML and fraud prevention platform
Experience the most intelligent AML and fraud prevention platform
Top AML Scenarios in ASEAN

The Role of AML Software in Compliance

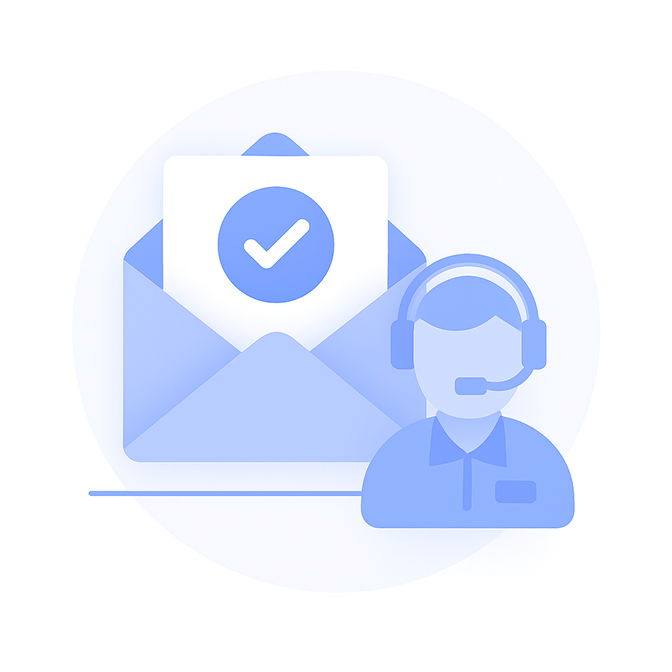