How Technology Can Ensure Effective AML Information Sharing
We are in a digital-first economy where goods and services delivery has moved significantly online. The availability of financial services related to investment, payments, money transfers and lending online has aided financial inclusion in a commendable manner -- thanks to the new-age fintech companies.
While the online financial services are helping us conduct financial activities in a faster, seamless and cost-effective way, there are increased possibilities of the abuse of digital financial infrastructure. Criminals are now doubly equipped to defraud customers and gain much more. At the same time, they are capitalizing on the loopholes of the current infrastructure and the lack of regulatory reforms to circulate their illicit money and stay away from the enforcement lens.
Special situations such as the COVID-19 pandemic have given more opportunities for criminals to adapt existing fraud schemes or create new ones. For instance, the US Department of Justice had uncovered numerous schemes where criminals obtained stimulus payments intended for individuals and companies with stolen or fake identities.
The story that has been influenced by the pandemic is being followed closely by many companies. The pandemic has affected anti-money laundering (AML) compliance within financial institutions, prompting them to think about new approaches and technologies to remain efficient and mitigate risk. Meanwhile, regulators across the globe are also pushing financial institutions to develop, test and implement AML compliance solutions based on new-age technologies such as artificial intelligence and machine learning.
{{ cta-first }}
Information sharing and AML compliance
Accurate, reliable and high-quality data is key to building AML compliance solutions based on machine learning. As money laundering techniques are growing in volume and complexity, we need more data inputs from varied data sources to differentiate good customers from bad customers. Financial institutions can no longer sustain fighting financial crimes the conventional way by just focussing on rules-based, siloed detection with no or limited insights from peer banks. AML insights from relevant stakeholders will help create a better context around customers and their activities.
Regulatory initiatives
Many regulators are working on creating a framework to share information between various stakeholders in the AML space. For example, the USA PATRIOT Act had provisions to enable greater information sharing among law enforcement, regulators and financial institutions regarding AML risks. Section 314(a) of the Act enables federal, state, local and European Union law enforcement agencies to reach out to US financial institutions through the US Treasury Department's Financial Crimes Enforcement Network (FinCEN) to locate accounts and transactions of persons that may be involved in terrorism or money laundering. Additionally, Section 314(b) provides a limited safe harbour for financial institutions to share information with one another in order to better identify and report potential money laundering or terrorist activities. There are similar approaches from the regulators in the UK, Australia, Singapore, Hong Kong, and Canada.
While we are not sure about the effectiveness of these initiatives, confidentiality and privacy requirements such as the Bank Secrecy Act (BSA) in the US and the General Data Protection Regulation (GDPR) in Europe are indeed barriers to information sharing. At the same time, advances in technology have brought in new approaches to AML information sharing without impacting privacy interests.
Read More: How AML Technology is Transforming Financial Crime Prevention
Use of technology in information sharing
Many technology innovations have compelling use cases in the area of AML information sharing. They can make AML programs more effective and efficient through enhanced information sharing while addressing privacy concerns. For example, distributed ledger technologies can be used to simplify financial sector responses to requests for information from the government under Section 314(a). Machine-learning technologies, which are proven to be effective in many AML use cases such as transaction monitoring, are encouraging a re-thinking of the types of information that can be shared among financial institutions for training transaction-monitoring algorithms.
Privacy-enhancing technology that can convert sensitive customer information into anonymous or pseudonymous attributes is also becoming widely available. This information can be used to train machine learning models and can create anomaly detection solutions with great accuracy. Federated Learning, a machine learning technique that trains an algorithm across multiple decentralized edge devices or servers holding local data samples without exchanging them, is another tool to leverage for effective transaction monitoring. The technology operates as a Hub-and-Spokes model where a machine learning algorithm, for instance, deep neural networks, is trained on multiple local datasets contained in local nodes without explicitly exchanging data samples.
Our revolutionary approach to AML information sharing
A regulatory technology company focused on AML, Tookitaki has developed a Federated Learning-enabled AML information sharing framework, titled the Typology Repository Management (TRM). Tookitaki has created an ecosystem of AML Knowledge through the Typology Repository (Hub) while breaking down silos through the AML Detection Engine (Spokes). Insights from the Hub can be seamlessly ingested through the Spokes by financial institutions to identify and prevent financial crime. Typology Repository is a fast-growing database of AML typologies or scenarios sourced from a network of AML experts globally, including financial institutions, law enforcement and regulators, and non-profit organizations. Typologies refer to patterns that are used to finance or launder money for illicit activities like drug trafficking, forced labour, forgery, terrorism, etc. They map varied customer activities that represent suspicious behaviour without using any Personally Identifiable Information (PII).
{{cta-ebook}}
Tookitaki Typology Repository is pre-packaged with Typology Developer Studio that allows the creation of typologies holistically through a No-Code user interface. Once created and verified, typologies can be downloaded by user institutions. Tookitaki AML engine – AMLS uses a proprietary AML insights language to deconstruct the typologies ingested from
Typology Repository into risk indicators and then generate automated thresholds based on customer risk levels. Finally, an inbuilt simulation engine validates typologies while using a maker-checker process to deploy them seamlessly.
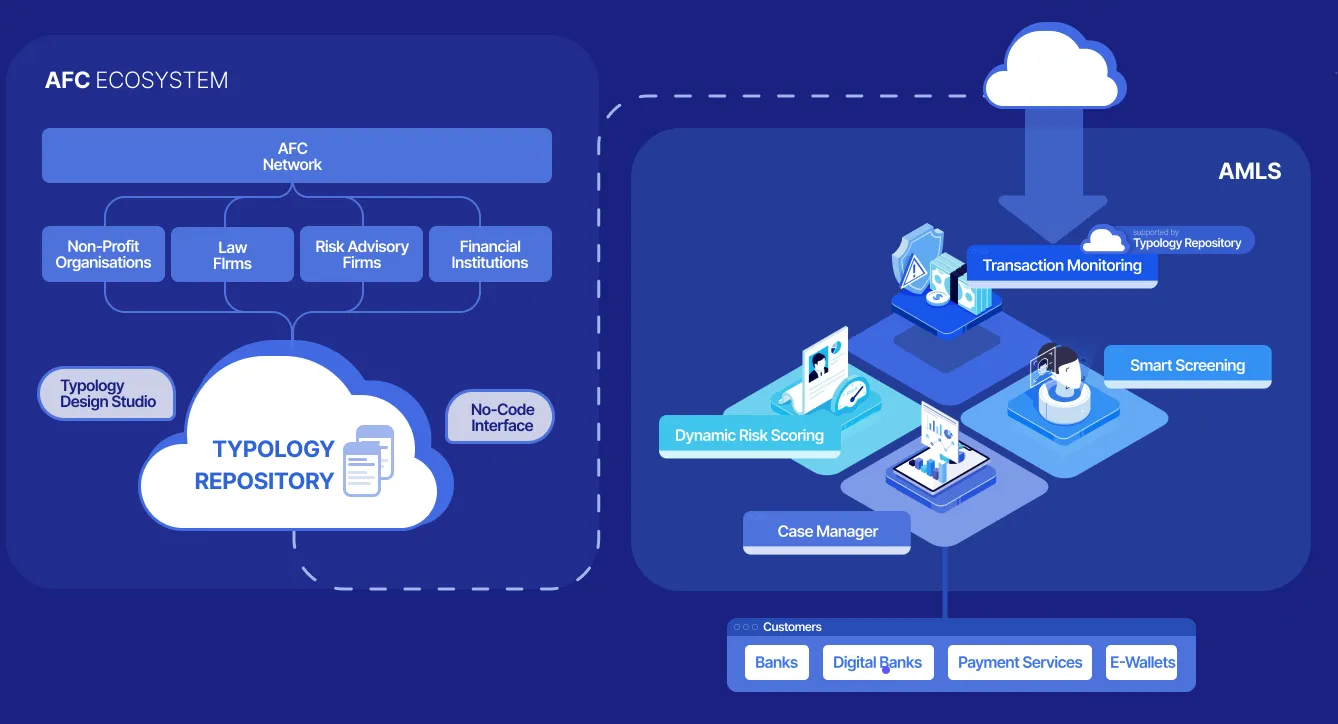
TRM enhances our machine learning-based transaction monitoring solution with superior detection capabilities. It is helping banks and fintech firms with financial crime identification and prevention by democratising AML insights through privacy-protected federated learning and precise detection through a hyper-configurable machine learning approach.
For more information on our solution and the ways in which it supercharges your transaction monitoring capabilities, please contact us.
Experience the most intelligent AML and fraud prevention platform
Experience the most intelligent AML and fraud prevention platform
Experience the most intelligent AML and fraud prevention platform
Top AML Scenarios in ASEAN

The Role of AML Software in Compliance

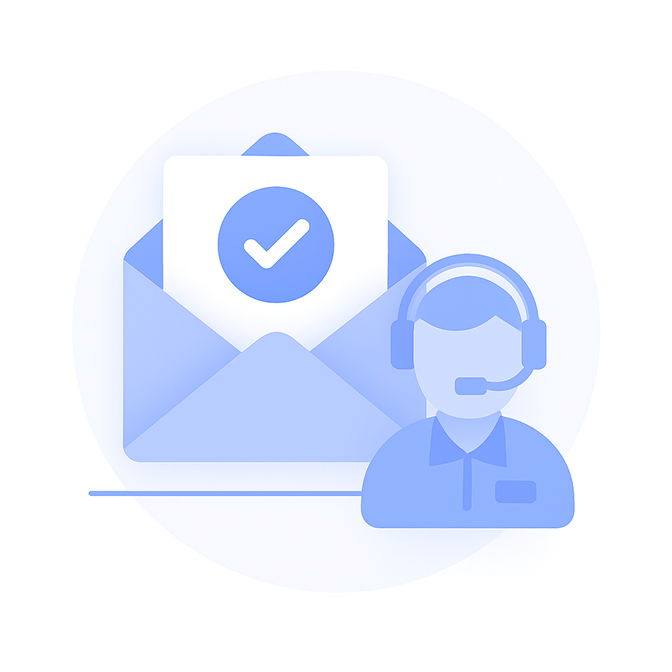